1 Introduction
Lubricants, whether extracted from unrefined petroleum (crude oil) or manufactured as synthetic oils, are vital elements in a broad spectrum of applications such as metalworking fluids, lubricating oils, internal combustion engines, gear oils, and transformer oils (Chari
et al.,
2012). Different automotive and industrial sources recently produce vast amounts of used lubricating oils worldwide which create serious environmental problems (Kupareva
et al.,
2013). One of the most efficient ways of managing waste lubricants is recycling and regeneration methods. Indeed, the efficient and effective recycling of used lubricants can be a great help for reduction of the environmental pollution; therefore, these methods will create high efficiency not only from the aspect of environmental friendliness but also from the viewpoint of financial and economic levels (Hsu
et al.,
2010).
Recycling of used oils has been developed in past fifty years, and modern techniques have been widely adopted and succeeded among the traditional approaches in commercial applications. Thus, with the rise in awareness of environmental and economic concerns as well as competitions in lubricant industries, one of the great challenges will be selecting and assessing the best available technology which can support industries, decision-makers, and regulators in directing environmental and economic concerns in industries with consideration of the application of reduction and prevention strategies (Chung
et al.,
2013).
For selection of an optimal recycling technology, various criteria should be considered. Hence, the selection process is a multi-criteria decision making (MCDM) problem. In a MCDM problem, decision maker encounters multiple alternatives affected by various criteria with beneficial or non-beneficial objective values (Parnell
et al.,
2013).
In past few years, several techniques have been developed to tackle MCDM problems such as the Multi-Objective Optimization on the Basis of Ratio Analysis (MOORA), the Technique for the Order of Prioritization by Similarity to Ideal Solution (TOPSIS), scoring models, outranking methods, and Axiomatic Design (AD) approach. Due to the complications of waste lubricant regenerative technologies, the selection of regenerative technology and strategy for used lubricant oil can be considered as a decision-analysis problem that is typically made by a committee of experts from academia, industry, and the government (Hsu
et al.,
2010).
In decision-making models, “risk” refers to undesirable various uncertain result of events. In some cases, the risk may be the measure of the degree of optimism about the information available in the problem (Hafezalkotob and Hafezalkotob,
2016a). Additionally, risk can be clarified as the intensity and probability of adverse effects in other cases (Ravindran
et al.,
2010). Like all decision-making scopes, in technology selection process, risk factors can emerge from different issues. For instance, risk factors may demonstrate the deviation between the nominal properties of technology provided in various handbooks (e.g. American Petroleum Institute (API) and United Nations (UN) compendiums for developing technologies) and the real properties of technology dependent on the design structure and experts review committee. Furthermore, risk factors may show the effect of the contrast between the designed and unexpected conditions (Hafezalkotob and Hafezalkotob,
2016a). The methods of defining risk factors for technology selection problems are explained in Section
3.4.
The Axiomatic Design (AD) approach is a major decision-making technique developed based on information theory and entropy principles by Suh (
1998). By integrating AD approach with fuzzy data, the Fuzzy Axiomatic Design (FAD) method is generated (Kulak and Kahraman,
2005a). By considering risk factors for each alternative with respect to criteria, Risk-Based Fuzzy Axiomatic Design (RFAD) method was developed (Kulak
et al.,
2015). For risk-based technology selection, the RFAD method can effectively be utilized. Entropy concept which is exploited in interdisciplinary fields (e.g. physical and social sciences such as economics and information theory) can be useful in the process of decision-making because it can evaluate deviation between data sets (Hafezalkotob and Hafezalkotob,
2016b).
The focus of the present paper is to introduce a decision-making approach for assessing the best available regenerative and recycling technology for lubricating oils. Adopting the appropriate technology can be a consequential challenge for investors and companies that have a developing strategy. This research examines five types of regenerative technologies that have already been frequently employed commercially and initiates a systematic technique for evaluating regenerative technologies. This research has two main steps: the first step is a decision-analysis considering all criteria and the second step is a categorized decision-analysis based on technical and economic characteristics.
This paper is structured as follows: Sections
2.1 and
2.2 respectively present a short survey on applications of MCDM methods in technology selection and AD approaches. Section
2.3 reviews waste lubricant oil regenerative technologies and Section
2.4 provides the research gap and contributions of current research. Section
3 gives a short explanation about Axiomatic Design (AD) approaches, Fuzzy Axiomatic Design (FAD), weighted FAD (WFAD), and FAD with risk factors (RFAD). Section
4 presents the applications of each approach in the technology selection for recycling used lubricant oils considering all criteria and categorized criteria, while Section
5 offers conclusions and recommendations for the future research.
4 Application of Axiomatic Design Approaches in a Waste Lubricant Oil Regenerative Technology Selection Problem
In this section, the FAD, WFAD, RFAD, and WRFAD approaches are utilized to select optimal regenerative technology for recycling waste oils into functional lubricating base oils. To reach a better understanding of regenerative technology selection problem and present a comprehensive analysis, technology selection is performed in two phases, i.e. first by considering all criteria, and second by considering categorized criteria which include technical and economic criteria.
As mentioned in Section
2.3, lubricating oil nowadays plays a vital role in various fields (Chari
et al.,
2012). With serious considerations for sustainability and environmental issues, selection of appropriate technology regarding key atributes is crucial. The degree of sustainability or the process stability in many technologies is low. On the other hand, many technologies are exploited around the world with the lack of concerns for environmental issues. Based on a report prepared by Iranian Industry Organization, there are more than 200 reprocessing units of waste oil in Iran which use acid/clay process. According to a statement of the United States Environmental Protection Agency (USEPA), acidic sludge which is the residue of acid/clay process is considered as a dangerous waste material (Jafari and Hassanpour,
2015).
One of the effective methods for decreasing the health and environmental threats of acidic sludge is neutralization through physical modifications. However, since neutralization process of acidic sludge is not economical considering the decrease in crude oil price in the past few years, many companies do not attempt to solve the problem. Technical and economic matters are two critical factors that every organization faces in selection process of an appropriate technology.
In the current study, based on the related researches (Hsu
et al.,
2010; Hsu and Liu,
2011; Chari
et al.,
2012; Jafari and Hassanpour,
2015) and the comments of experts, the following five technologies are determined as candidate alternatives: (a) Acid-clay process, (b) Acid-activated clay process, (c) Thin/wiped film evaporation (based on vacuum distillation), (d) Solvent extraction process, and (e) Hydro-process (Hydro-extraction). A short graphical summary of the candidate technologies is shown in Fig.
4.
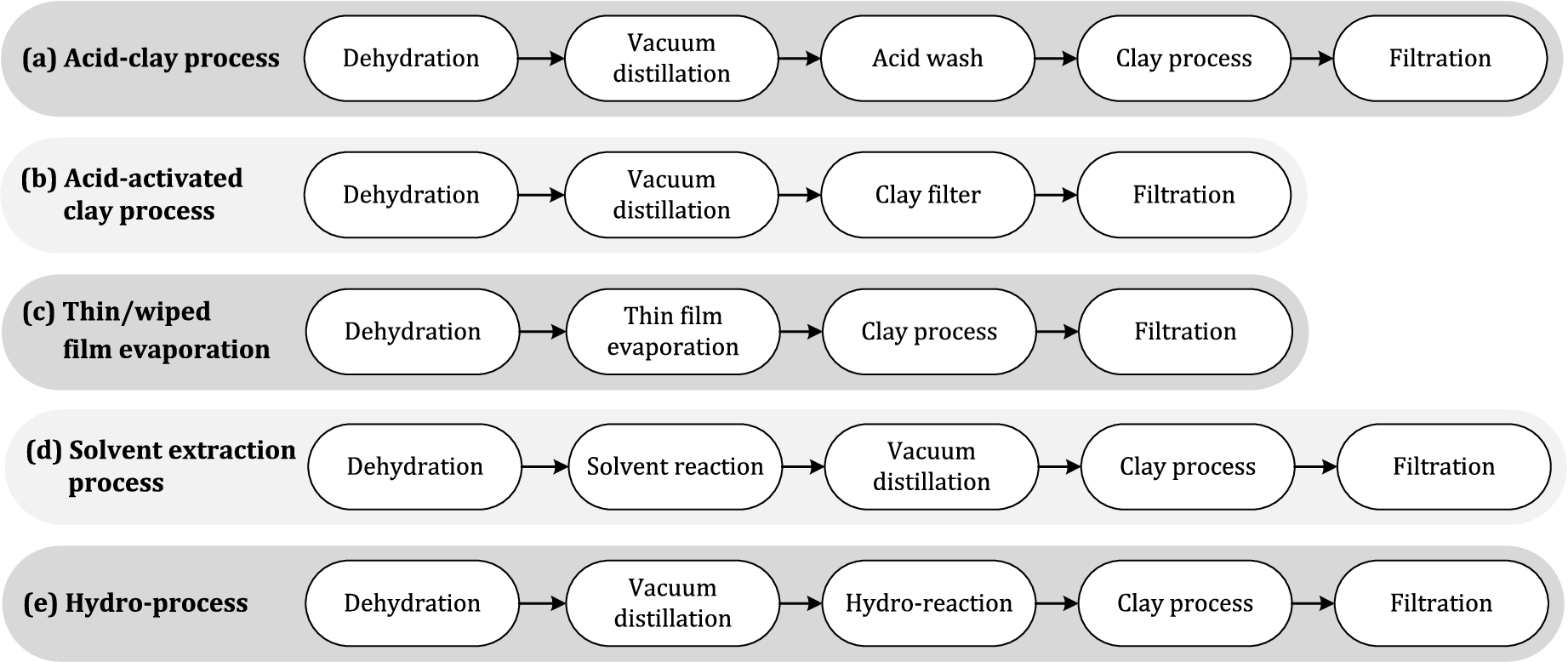
Fig. 4
A typical illustration of the steps of candidate technologies.
The focus of this study is on popular and practical regenerative technologies for waste lubricant oils; however, other technologies exist which are in research and development state or utilized in few numbers of plants such as KTI process, Safety–Kleen technology, Axens/Viscolube (REVIVOIL) technology, IFP technology/Snamprogetti technology, HyLube process, BERC/NIPER hydrogenation, and Vaxon Process (Chari
et al.,
2012). In this practical green technology selection problem, thirteen criteria are specified based on various researches (Dalla
et al.,
2003; Hsu
et al.,
2010; Hsu and Liu,
2011; Chari
et al.,
2012; Kupareva
et al.,
2013; Mohammed
et al.,
2013; Jafari and Hassanpour,
2015) and the comments of experts. Table
1 presents the evaluation criteria in decision process for used lubricant oil regenerative technologies classified into three aspects.
Table 1
Criteria definition for selection of waste lubricant oil regenerative technologies.
Aspects |
ID∗
|
Criteria |
Description |
Technicality and sustainability |
T1 |
Compatibility with all types of used oil |
The availability of water content, organic and chemical contaminants (e.g. Paraffin contaminants, PCB contaminants, and heavy metals), light lubricants (e.g. diesel and solvents), and sludge or crude oil within the used oil which affects the margin of production with diverse technologies |
|
T2 |
Quality of product |
Based on laboratory tests provided by American Society for Testing and Materials (ASTM) and with reference to American Petroleum Institute (API) which classify base-oil quality into five groups, quality of the product is determined (American Petroleum Institute, 2015) |
|
T3 |
Proven technology and adaptability for future |
Due to the application of every technology, it is substantial to identify the type used in various scenarios (i.e. commercially available, prototype, research and development state). Based on the different states of the technology and its adaptability to be upgraded, experts can rank technologies |
|
T4 |
Process sustainability and stability |
It is crucial for every production plant to have stable and sustainable operations which results in the desired consequences. The technology should be applicable in variable situations. Besides, risk estimation and real-time problem solving are essential |
|
T5 |
Degree of automation/ sophistication |
Knowledge and human resource managements are critical factors in every industry and organization. The level of sophistication of technologies is based on the degree of knowledge and availability of the human resource. Subsequently, the level of automation is a critical factor in the efficiency of production |
Health, safety and environmental considerations |
E1 |
Risk levels regarding the environment, e.g. as for lands, ecological receptors, and water contaminations |
Chemical and physical contaminants are one of the most stressors to ecosystems and the environment which can induce an adverse and irreversible effects. Regenerating plants may increase risk levels regarding the nature by producing specific by-products, e.g. acidic sludge and water contaminations |
|
E2 |
Risk levels for workers, communities, and beneficiaries |
One of the fundamental requirements for selecting a technology is the level of influence on employees and communities. It is crucial to choose a technology to decrease risk among beneficiaries of any production process |
|
E3 |
Energy and water consumption |
Every technology has variable intake of energy and water. The optimal technology minimizes the consumption of energy and water |
|
E4 |
Emissions and odor (pollution) |
Decomposition of chemical components by heat and solvents may cause atmospheric pollutions. Volatile Organic Compounds (VOC) which easily evaporate are very common in processing hydrocarbons. In optimal operations, pollution rate is minimized |
Economic considerations |
S1 |
Income generation potential |
Dependent on the production method, level of technology, production economic standard parameters, production margin profit of a specific technology is obtained |
|
F1 |
Capital costs/ investment costs |
Based on the feasibility evaluation of a technology which provides investment information, e.g. fixed and variable costs, the expenses of a technology are determined |
|
F2 |
Operation and maintenance costs |
Operation costs are defined according to the infrastructures of the production plant, specific material handling, and the total maintenance cost of a technology. Also, the depreciation rate of any technology has a direct influence on this criterion |
|
F3 |
Economic viability |
An economically viable technology is feasible, innovative, and sustainable regarding investing resources. A technology is more feasible when its predetermined goals are achieved faster, i.e. by reaching the head to head point |
Table 2
General risk factors regarding waste lubricant oil regenerative technologies.
Aspects |
Risk label |
Description |
Characteristics and limitations of raw materials |
-
• Physical contaminations (e.g. water content, light hydrocarbons, heavy metals, and synthetic oils)
-
• Chemical contaminations (e.g. paraffin, PCB, and PAO)
-
• Physical and chemical properties of raw material (e.g. viscosity, flash point, pour point, and colour)
-
• Availability of raw material
-
• Transportation of raw material
|
There are a couple of limitations considering raw materials. Mentioned risk factors are some of the influencing factors which can make impact on elements such as quality and sustainability of finished product or may have environmental consequences |
Characteristics of finished product (i.e. base-oils) |
-
• Physical contaminations (e.g. anonymous particles, water content, bleaching clay particles, and heavy metals)
-
• Chemical contaminations (e.g. sulfonate and paraffin)
-
• Physical and chemical properties of finished product (e.g. viscosity, flash point, odour, pour point, and colour)
|
The existence of contaminations and low standard of technical properties in finished product will result in poor quality of product and increases environmental and health parameters |
Technological complexities |
-
• Technical knowledge (e.g. technological and operational knowledge)
-
• Manufacturing method (e.g. batch and continuous production)
-
• Compatibility with existing production plant
-
• Process stability
-
• Adaptability to future situations
-
• Level of technical sophistication
|
Flexibility and adaptability of infrastructures and the existing technologies are important factors in creation of technical complexities. Technical knowledge of sophisticated technologies has a huge impact on risk prevention and optimization of process stability |
Human resource factors |
-
• Employees performance and influencing elements such as employees satisfaction and loyalty
-
• Compatibility and suitability of technology regarding the existing educational and skill levels
-
• Human reliability
-
• Availability of experts in the required fields
-
• Level of employees efficiency
-
• Recruitment factors
|
Human resource is one of the valuable assets of organizations. One of the great responsibilities of industrial plants is to optimize effectiveness and efficiency of resources. Human resource factors can have effective influence on quality and quantity of products |
Waste management |
|
Hazardous dumps and leakage of specified wastes can have irreparable damages to the environment. Today, one the most important factors of chemical regenerative technologies is waste management. The considerable amount of hazardous wastes will make this factor a great threat to factories |
Topographical and geographical factors |
|
Topographical and geographical aspects such as location and physical space can have great effects on quantity of products dependent on the size of operation |
Health, safety and environmental issues (HSE factors) |
-
• Human resource safety factors such as on-site and off-site and insurance
-
• Force major health and safety conditions for employees
-
• Greenhouse and non-greenhouse gases
-
• By-product emission and odour (e.g. light combustible hydrocarbon gasses)
-
• Incident prevention (e.g. hazardous gas leakage, fire, explosion, and electrical shocks)
-
• Ergonomics (e.g. employees fatigue reduction systems, increasing safety spots, and noise reduction)
|
Dependent on the scale and sensitivity of the proposed technological interventions, a full-fledged risk assessment exercise is mandatory in HSE factors. Before making decision on the final technology enhancement, it is crucial to analyse health, safety, and ergonomic measures for employees and environmental issues for biodiversity and humans |
Specific energy and material consumption |
-
• Specific energy usage (e.g. water, gas, fuel, and electricity)
-
• Specific material consumption (e.g. hydrogen, polymer, various solvents, and bleaching clay)
|
Consuming specific energies or materials may cause overhead expenses and inefficient technology |
Economic factors and regulations |
-
• Entrepreneurship
-
• Economic investor factors
-
• Cultural factors
-
• Depreciation and maintenance factors
-
• Revenue and marginal factors
-
• Competitiveness and strategies
-
• Scheduling and resource factors
-
• International and domestic regulations and rules
|
The main reason for all measures which organizations adopt to enhance process sustainability and improve quality and quantity is to gain a high marginal profit from the whole process. Economic factors may pose high risks to an organization |
Table 3
Specific risk factors regarding waste lubricant oil regenerative technologies.
Risk label |
Influenced technologies |
Description |
Paraffin contamination |
(a), (b), (c), (d) |
Mixing used oil with all sorts of materials (e.g. sludge from oil tankers carrying crude oil) causes finished product to be misty and cloudy even after regeneration process. High temperatures (over 340${^{\circ }}$C) can reduce paraffin whereas the reduction amount can vary in different technologies |
Coke formation |
(a), (b), (c), (d), (e) |
High temperatures in regenerative technologies due to nature of hydrocarbons result in coke formation (from cracked or decomposed cracked oil) and in any regenerative process, coke formation is inevitable in some units (e.g. reboilers, distillation towers, and reactors surface). Coke formation causes blockage in valves, pipes, and all the reactors in the surface |
Oxidation |
(a), (b) |
Application of sulfuric acid and high crack temperature cause the finished product, i.e. base oil, to oxidize and affect the colour of the base oil |
High temperature cracking |
(a), (b) |
Existence of high temperature in distillation column or in clay mixing reactor not only causes a lot of coke formation, but also, reduces the yield of the finished product and produces huge amount of cracked gasses |
Sulfuric acid purity |
(a) |
The purity of the applied sulfuric acid is a critical factor in acid-wash process and exerts remarkable effects on the sludge formation in reactors |
Type of clay |
(a), (b) |
In regeneration process, a non-activated clay needs small amount (3–4%) of sulfuric acid and high temperature oil in reactor. On the other hand, an activated clay works well in much lower temperature with the same result but higher prices |
Quantity of clay |
(b), (c) |
Using about 40–45% of non-activated clay (e.g. bentonite clay) in clay process not only produces huge amount of waste and disposed clay, but also, reduces the product margin due to oil content in the used clay |
High level of fatigue and corrosion in process |
(a) |
Presence of sulfuric acid in various forms in regeneration process causes corrosion in the whole production system in contact with acid. Existence of acid in any process unit has decay effects on whole process unit and increases the final depreciation rate |
Continuous maintenance service |
(a), (b), (c), (d), (e) |
One of the essential factors in every operating plant is preventive maintenance. Regarding high temperature within the regenerative technologies, regular maintenance to safe-guard the operation is crucial. Subsequently, the acidic and bleaching plants need higher attention for maintenance |
Acidic sludge storage |
(a) |
According to high remaining acid contents and salts within acid-clay process, it is important to have a secure handling and material storage facility. In case of spillage or storage failure, the excess acid within sludge causes the sludge to be runny. Therefore, in case of dumping sludge material in dead land, it would not penetrate and if covered by soil it would surface eventually |
Spent clay storage |
(a), (b) |
Used clay should be stored under a covered area because a small amount of oil exists in it. Besides, this measure helps in blocking direct sun contact as to avoid evaporation of remaining oil within the spent clay. Moreover, correct storage would prevent contamination of the underground water and surface running water |
Sulfuric acid procurements |
(a), (b) |
Transportation of sulfuric acid in any type of container needs special precaution and safety because of its hazardous nature. This element is a very important factor in particular processes such as acid-clay process and acid-activated clay process |
Employees training for application of sulfuric acid |
(a) |
Because of the hazardous nature of sulfuric acid, it is crucial to have a training process for employees to deal with the material |
Employees training for application of bleaching clay |
(b) |
High quantity of clay usage in acid-activated clay process may cause troubles for employees. Specific training courses for handling the clay (e.g. feeding and discharging methods) can boost the effectiveness of bleaching process |
Acid handling |
(a), (b) |
Sulfuric acid is a highly corrosive; thus, particular attentions should be given throughout the transportation and consumption process |
Clay handling |
(a), (b), (c), (d) |
As partial hazardous material, bentonite clay should be handled with care and protection. Because of small particles (micro-particles) in bentonite clay, it would harm employees without adopting proper safety measures |
Fire eruption |
(a), (b), (c), (d), (e) |
Presence of high operating temperature in lubricant oil regenerative plant besides light fuels and cracked gasses create a high-risk environment for fire eruptions. Employees training, proper fire prevention, and disarming systems reduce the probability of fire eruptions. In hydro-process plant, the use of hydrogen provides an extremely high-risk environment |
Human errors in maintenance overhaul |
(a), (b) |
Regarding the high demand of maintenance in acid-clay process and acid-activated clay process, probability of human errors is high. High temperature and huge amount of clay usage within the reactors can have negative impacts on employees’ safety and production process |
Cracked gasses (Gas emission) |
(a), (b), (c), (d), (e) |
There is a certain amount of light oils (e.g. diesel and petrol) in used oils which evaporate during the operating process. The process produces cracked gasses (e.g. VOCs). By using incinerators, these gasses can be burned in high temperature and gas emission would be reduced |
Acidic salt emission |
(a) |
In the sulfonating unit and clay reactors, different sorts of acidic salts (e.g. sulfonates) form during the acid clay process. The process has a huge amount of air pollution which causes immediate breathing problems. The mentioned units besides a neutralization unit help in minimizing the acidic salt |
Water emission |
(a), (b), (c), (d), (e) |
Presence of water content in used oil is inevitable. This water content should be evaporated and condensed separately as not to vaporize to atmosphere. There is an amount of water content mixed with light oil which should be separated by any means before disposing to drain. Since there is also about 7% water in clay packing, the more clay consumption, the more water within the reactor will be and consequently the more water evaporates which should be treated prior to drain disposal. The waste water in case of drainage in sanitation (ego) system would result in oil contamination in the sewage plant. In case of dumping sludge and used oils at the ground, they would contaminate the underground water sources |
Acidic sludge emission |
(a) |
Due to high consumption of sulfuric acid in acid clay process up to 25% of initial oil in volume, it should be treated carefully. Regarding hazardous nature of acidic sludge, this acidic waste should be neutralized by mixing acidic sludge with casting soda or hydroxide calcium prior to disposing or any other usage |
Clay consumption |
(a), (b), (c), (d) |
To improve the colour of finished base-oil and clarify of finished product, earth clay as active or bentonite forms is applied. High consumption of clay may have disposal and storage problems. The waste clay which produces by regeneration process can be sent to cement factories where it can be utilized as filler to cement clinker |
Fuel consumption |
(a), (b), (c), (d), (e) |
Fuel is employed to generate high temperature for regenerative operations. Higher fuel consumption has higher environmental impacts such as heat exchange to greenhouse and gas emissions |
Caustic soda lye handling and process |
(a) |
In acid clay process, using caustic soda lye as a neutralizing agent is compulsory because of the active sulfuric acid within the process. Sodium hydroxide as a hazardous chemical has destructive effects on human skin and particularly eyes. Also, if sodium hydroxide penetrates to the ground, it would contaminate underground water and vegetation |
Quality of solvent |
(d), (e) |
Quality of solvent significantly affects the production result. Low-quality solvent not only reduces the margin of the product but also consumes more energy in the specific units, e.g. compressors, of the regenerative process |
Purity of hydrogen |
(e) |
One of the important elements in hydro-process is purity of hydrogen which can affect the hydro-process operation and either increase or reduce the quantity and quality of finished product |
Catalyst type |
(e) |
The type of catalyst used in hydro-process massively affects the process by prolonging the time of process or it can reduce process time and affects the marginal yield of production |
Vacuum failure |
(c) |
The thin/wiped film evaporation process mainly runs by high vacuum either using vacuum pumps or ejectors. Any failure causes the unprocessed oil to drop to the receivers and ruin the oil and increase the pressure within the reactor which is dangerous and can lead to fire and gas emission in the operation plant |
Solvent leakage |
(d), (e) |
Solvents are low flash point substances; thus, a leakage may cause fire or explosion in the unit and can have a catastrophic effect on the operation site |
Specific technical knowledge |
(c), (d), (e) |
Lack of knowledge in any sophisticated process may cause enormous damages. Special trainings are needed to be executed for all employees in case of emergency |
Operating system failure |
(c), (d), (e) |
Due to the sophisticated operations and systems, failure of any operational procedure will result in a catastrophic disaster such as huge explosions |
Solvent storage |
(d), (e) |
Regarding the low flash point of solvent, serious attention and special storage are required |
Risk factors for technology selection problems can be defined differently dependent on the nature of efficient technologies. In this practical case, two categories of risk factors have been defined for each technology and a comprehensive description of each element is presented. The first category introduces the general risk factors which have a roughly similar effect on all waste lubricant oil regenerative technologies. The second category presents specific risk factors that may differently affect the technologies. Tables
2 and
3 list the general and specific risk factors, respectively.
In this case study, the ratings of candidate technologies on each criterion are linguistic variables obtained based on comments of experts. Table
4 demonstrates the linguistic variables and their corresponding triangular fuzzy sets.
Table 4
Linguistic terms and the corresponding triangular fuzzy numbers.
Linguistic term |
Abbreviated linguistic term |
Triangular fuzzy number |
Extremely Low |
EL |
$(0,1,2)$ |
Very Low |
VL |
$(1,2,3)$ |
Low |
L |
$(2,3,4)$ |
Medium Low |
ML |
$(3,4,5)$ |
Medium |
M |
$(4,5,6)$ |
Medium High |
MH |
$(5,6,7)$ |
High |
H |
$(6,7,8)$ |
Very High |
VH |
$(7,8,9)$ |
Extremely High |
EH |
$(8,9,10)$ |
According to the thirteen criteria, candidate technologies, and the conversation table for linguistic variables, the decision matrix has been structured as shown in Table
5. The functional requirement for each criterion is obtained by experts’ comments. Therefore, for every criterion, a design range is determined which is then transformed into triangular fuzzy numbers based on Table
4. System ranges are the translated form of the linguistic ratings of candidate technologies on each criterion specified through the comments of experts. Table
5 also provides subjective significance coefficients.
Table 5
System and design ranges in the form of triangular fuzzy numbers.
Criteria |
Alternatives of waste lubricant oil regenerative technology |
Subjective significance coefficients |
Design ranges |
Acid-clay process |
Acid-activated clay process |
Thin/wiped film evaporation |
Solvent extraction process |
Hydro-process |
T1 |
$(6,7,8)$ |
$(6,7,8)$ |
$(7,8,9)$ |
$(6,7,8)$ |
$(6,7,8)$ |
0.084 |
$(7,8,9)$ |
T2 |
$(6,7,8)$ |
$(6,7,8)$ |
$(7,8,9)$ |
$(7,8,9)$ |
$(7,8,9)$ |
0.087 |
$(7,8,9)$ |
T3 |
$(4,5,6)$ |
$(4,5,6)$ |
$(6,7,8)$ |
$(5,6,7)$ |
$(6,7,8)$ |
0.068 |
$(5,6,7)$ |
T4 |
$(4,5,6)$ |
$(4,5,6)$ |
$(6,7,8)$ |
$(4,5,6)$ |
$(6,7,8)$ |
0.068 |
$(5,6,7)$ |
T5 |
$(4,5,6)$ |
$(4,5,6)$ |
$(6,7,8)$ |
$(6,7,8)$ |
$(6,7,8)$ |
0.052 |
$(5,6,7)$ |
E1 |
$(7,8,9)$ |
$(7,8,9)$ |
$(5,6,7)$ |
$(5,6,7)$ |
$(5,6,7)$ |
0.084 |
$(6,7,8)$ |
E2 |
$(4,5,6)$ |
$(4,5,6)$ |
$(5,6,7)$ |
$(5,6,7)$ |
$(5,6,7)$ |
0.073 |
$(5,6,7)$ |
E3 |
$(4,5,6)$ |
$(4,5,6)$ |
$(5,6,7)$ |
$(5,6,7)$ |
$(5,6,7)$ |
0.054 |
$(4,5,6)$ |
E4 |
$(7,8,9)$ |
$(7,8,9)$ |
$(5,6,7)$ |
$(5,6,7)$ |
$(5,6,7)$ |
0.089 |
$(6,7,8)$ |
S1 |
$(5,6,7)$ |
$(5,6,7)$ |
$(6,7,8)$ |
$(5,6,7)$ |
$(5,6,7)$ |
0.090 |
$(6,7,8)$ |
F1 |
$(3,4,5)$ |
$(3,4,5)$ |
$(4,5,6)$ |
$(5,6,7)$ |
$(5,6,7)$ |
0.091 |
$(4,5,6)$ |
F2 |
$(5,6,7)$ |
$(5,6,7)$ |
$(4,5,6)$ |
$(5,6,7)$ |
$(5,6,7)$ |
0.064 |
$(4,5,6)$ |
F3 |
$(4,5,6)$ |
$(4,5,6)$ |
$(6,7,8)$ |
$(5,6,7)$ |
$(5,6,7)$ |
0.096 |
$(5,6,7)$ |

Fig. 5
Common area of ${\tilde{x}_{11}}$ and ${\tilde{d}_{1}}$.
System area denotes the area under the membership function of system range which in current data sets is the area of the triangle. Common area is calculated utilizing Eq. (
7). Fig.
5 illustrates the common and system areas related to design and system ranges
${\tilde{x}_{11}}$ and
${\tilde{d}_{1}}$.
Common area
${c_{11}}$ and system area
${\hat{x}_{11}}$ are computed as follows:
Common areas for the problem are reported in Table
6. In this technology selection problem, the values of system areas are all the same value, i.e. 1. That is, the height of the triangle of all system ranges is 1 and because of the considered linguistic variables, the base of the triangle is always 2 which leads to the system area equal to 1.
Table 6
Common areas in the waste lubricant oil regenerative technologies selection problem.
Criteria |
Alternatives of waste lubricant oil regenerative technology |
Acid-clay process |
Acid-activated clay process |
Thin/wiped film evaporation |
Solvent extraction process |
Hydro-process |
T1 |
0.25 |
0.25 |
1 |
0.25 |
0.25 |
T2 |
0.25 |
0.25 |
1 |
1 |
1 |
T3 |
0.25 |
0.25 |
0.25 |
1 |
0.25 |
T4 |
0.25 |
0.25 |
0.25 |
0.25 |
0.25 |
T5 |
0.25 |
0.25 |
0.25 |
0.25 |
0.25 |
E1 |
0.25 |
0.25 |
0.25 |
0.25 |
0.25 |
E2 |
0.25 |
0.25 |
1 |
1 |
1 |
E3 |
1 |
1 |
0.25 |
0.25 |
0.25 |
E4 |
0.25 |
0.25 |
0.25 |
0.25 |
0.25 |
S1 |
0.25 |
0.25 |
1 |
0.25 |
0.25 |
F1 |
0.25 |
0.25 |
1 |
0.25 |
0.25 |
F2 |
0.25 |
0.25 |
1 |
0.25 |
0.25 |
F3 |
0.25 |
0.25 |
0.25 |
1 |
1 |
The triangular fuzzy system ranges of Table
5 can be defuzzified using Eq. (
16) to obtain the crisp values needed for calculating entropy significance coefficients. After obtaining defuzzified numbers, normalized values are computed using Eq. (
12). The normalized values of the defuzzified system ranges are shown in Table
7. Regarding Eq. (
13), Shannon entropy measures are obtained for the criteria of the problem. The entropy measures assist in specifying more critical criteria by dispersion analysis of the decision matrix. Eq. (
14) is utilized to calculate objective significance coefficients. Finally, the subjective and objective significance coefficients are integrated based on Eq. (
15). Table
8 shows the objective significance coefficients and the integrated entropy significance coefficients for the current green technology selection problem.
Table 7
Normalized defuzzified system ranges.
Criteria |
Alternatives of waste lubricant oil regenerative technology |
Acid-clay process |
Acid-activated clay process |
Thin/wiped film evaporation |
Solvent extraction process |
Hydro-process |
T1 |
0.194 |
0.194 |
0.222 |
0.194 |
0.194 |
T2 |
0.184 |
0.184 |
0.211 |
0.211 |
0.211 |
T3 |
0.167 |
0.167 |
0.233 |
0.200 |
0.233 |
T4 |
0.172 |
0.172 |
0.241 |
0.172 |
0.241 |
T5 |
0.161 |
0.161 |
0.226 |
0.226 |
0.226 |
E1 |
0.235 |
0.235 |
0.176 |
0.176 |
0.176 |
E2 |
0.179 |
0.179 |
0.214 |
0.214 |
0.214 |
E3 |
0.179 |
0.179 |
0.214 |
0.214 |
0.214 |
E4 |
0.235 |
0.235 |
0.176 |
0.176 |
0.176 |
S1 |
0.194 |
0.194 |
0.226 |
0.194 |
0.194 |
F1 |
0.160 |
0.160 |
0.200 |
0.240 |
0.240 |
F2 |
0.207 |
0.207 |
0.172 |
0.207 |
0.207 |
F3 |
0.172 |
0.172 |
0.241 |
0.207 |
0.207 |
Table 8
Entropy measures, objective and integrated significance coefficients.
|
T1 |
T2 |
T3 |
T4 |
T5 |
E1 |
E2 |
E3 |
E4 |
S1 |
F1 |
F2 |
F3 |
${E_{j}}$ |
0.999 |
0.999 |
0.994 |
0.991 |
0.992 |
0.994 |
0.998 |
0.998 |
0.994 |
0.999 |
0.990 |
0.998 |
0.995 |
${w_{j}^{o}}$ |
0.015 |
0.021 |
0.113 |
0.142 |
0.130 |
0.104 |
0.040 |
0.040 |
0.104 |
0.020 |
0.163 |
0.025 |
0.084 |
${w_{j}^{\ast }}$ |
0.017 |
0.024 |
0.101 |
0.126 |
0.089 |
0.113 |
0.038 |
0.028 |
0.120 |
0.024 |
0.194 |
0.021 |
0.105 |
As described in Section
3.2, the probability of achieving design range
${p_{ij}}$ and the information content
${I_{ij}}$ for the technology selection problem are respectively calculated employing Eqs. (
8) and (
9). Table
9 demonstrates information contents of the FAD approach. Some of the information contents in Table
9 equal zero, that is, the corresponding system and common areas are equal
t (e.g.
${\tilde{d}_{1}}={\tilde{x}_{81}}\to {c_{81}}={\hat{x}_{81}}\to {I_{81}}=0$). In Table
9, information content equal to two denotes that the system area is fourfold the common area (e.g.
${c_{11}}={\hat{x}_{11}}/4\to {I_{11}}=2$). In AD principles, when information content tends to infinity, common area equals zero. In other words, the system and design ranges are not overlapped. In this case, which an information content is infinity, the corresponding total information content will also be calculated as infinity and the candidate technology will be the worst option. Regarding the integrated significance coefficients
${w_{j}^{\ast }}$, i.e. Eq. (
15), the information contents
${I_{ij}^{{w^{\ast }}}}$ are determined employing Eq. (
17).
Table 9
Information contents of the FAD method.
Criteria |
Alternatives of waste lubricant oil regenerative technology |
Acid-clay process |
Acid-activated clay process |
Thin/wiped film evaporation |
Solvent extraction process |
Hydro-process |
T1 |
2 |
2 |
0 |
2 |
2 |
T2 |
2 |
2 |
0 |
0 |
0 |
T3 |
2 |
2 |
2 |
0 |
2 |
T4 |
2 |
2 |
2 |
2 |
2 |
T5 |
2 |
2 |
2 |
2 |
2 |
E1 |
2 |
2 |
2 |
2 |
2 |
E2 |
2 |
2 |
0 |
0 |
0 |
E3 |
0 |
0 |
2 |
2 |
2 |
E4 |
2 |
2 |
2 |
2 |
2 |
S1 |
2 |
2 |
0 |
2 |
2 |
F1 |
2 |
2 |
0 |
2 |
2 |
F2 |
2 |
2 |
0 |
2 |
2 |
F3 |
2 |
2 |
2 |
0 |
0 |
In the current study, there are two types of risk factors, i.e. general and specified risk factors, which are respectively explained in Tables
2 and
3. The most of general risk aspects affect all criteria in the same amount, thus their corresponding risk factors are mainly equal to zero in risk measurements which means the specified element does not have any risk influence on the ratings of technologies on the criteria. For example, investor and scheduling factors would influence all technologies identically. Lack of timely schedule or finance resource would affect technology selection intrinsically and it does not matter which technology is selected when there is a lack of sponsorship. The risk factors, shown in Table
10, are the aggregate values of the general and specified risk factors for the problem. Using these risk factors, information contents of RFAD method
${I_{ij}^{r}}$ are computed based on Eq. (
20) which are shown in Table
11.
Table 10
Proposed risk factors for the regenerative waste lubricant technologies.
Criteria |
Alternatives of waste lubricant oil regenerative technology |
Acid-clay process |
Acid-activated clay process |
Thin/wiped film evaporation |
Solvent extraction process |
Hydro-process |
T1 |
0.335 |
0.350 |
0.090 |
0.090 |
0.100 |
T2 |
0.150 |
0.233 |
0.075 |
0.075 |
0.050 |
T3 |
0 |
0 |
0 |
0 |
0 |
T4 |
0.288 |
0.275 |
0.080 |
0.205 |
0.535 |
T5 |
0.050 |
0.040 |
0.235 |
0.217 |
0.313 |
E1 |
0.356 |
0.317 |
0.175 |
0.137 |
0.250 |
E2 |
0.172 |
0.236 |
0.070 |
0.113 |
0.357 |
E3 |
0 |
0 |
0 |
0 |
0 |
E4 |
0.350 |
0.285 |
0.050 |
0.055 |
0.055 |
S1 |
0 |
0 |
0.150 |
0.270 |
0.380 |
F1 |
0 |
0 |
0 |
0 |
0 |
F2 |
0 |
0 |
0 |
0 |
0 |
F3 |
0 |
0 |
0 |
0 |
0 |
Table 11
Information contents of the RFAD method.
Criteria |
Alternatives of waste lubricant oil regenerative technology |
Acid-clay process |
Acid-activated clay process |
Thin/wiped film evaporation |
Solvent extraction process |
Hydro-process |
T1 |
2.589 |
2.621 |
0.136 |
2.136 |
2.152 |
T2 |
2.234 |
2.383 |
0.112 |
0.112 |
0.074 |
T3 |
2 |
2 |
2 |
0 |
2 |
T4 |
2.489 |
2.464 |
2.120 |
2.331 |
3.105 |
T5 |
2.074 |
2.059 |
2.386 |
2.352 |
2.542 |
E1 |
2.635 |
2.549 |
2.278 |
2.212 |
2.415 |
E2 |
2.272 |
2.388 |
0.105 |
0.172 |
0.636 |
E3 |
0 |
0 |
2 |
2 |
2 |
E4 |
2.621 |
2.484 |
2.074 |
2.082 |
2.082 |
S1 |
2 |
2 |
0.234 |
2.454 |
2.690 |
F1 |
2 |
2 |
0 |
2 |
2 |
F2 |
2 |
2 |
0 |
2 |
2 |
F3 |
2 |
2 |
2 |
0 |
0 |
Based on the information contents of the RFAD approach
${I_{ij}^{r}}$ and the integrated significance coefficients, the information contents of the WRFAD method are computed using Eqs. (
23). Total information contents for the FAD, WFAD, RFAD, WRFAD techniques considering all criteria are respectively calculated by applying Eqs. (
10), (
18), (
21), and (
24) which have been shown in Table
12.
Table 12
Total information contents considering all criteria.
Alternatives of waste lubricant oil regenerative technology |
Total information contents |
FAD |
WFAD |
RFAD |
WRFAD |
Acid-clay process |
24 |
12.702 |
26.915 |
12.818 |
Acid-activated clay process |
24 |
12.702 |
26.949 |
12.813 |
Thin/wiped film evaporation |
14 |
7.491 |
15.446 |
7.537 |
Solvent extraction process |
18 |
9.530 |
19.852 |
9.591 |
Hydro-process |
20 |
10.603 |
23.696 |
10.725 |
Table
13 indicates the rankings of the technology selection problem considering all criteria. The optimal technology is specified by minimizing the corresponding total information contents. Dependent on Eqs. (
11), (
19), (
22), and (
25), the optimal technology for regenerating waste lubricant oils is
${A_{\mathrm{FAD}}^{\ast }}={A_{\mathrm{WFAD}}^{\ast }}={A_{\mathrm{RFAD}}^{\ast }}={A_{\mathrm{WRFAD}}^{\ast }}$ = thin/wiped film evaporation technology.
Table 13
Rankings of the candidate technologies considering all criteria.
Alternatives of waste lubricant oil regenerative technology |
Rankings |
FAD |
WFAD |
RFAD |
WRFAD |
Acid-clay process |
4 |
4 |
4 |
5 |
Acid-activated clay process |
4 |
4 |
5 |
4 |
Thin/wiped film evaporation |
1 |
1 |
1 |
1 |
Solvent extraction process |
2 |
2 |
2 |
2 |
Hydro-process |
3 |
3 |
3 |
3 |
As aforementioned in the beginning of Section
4, the same problem can be tackled by considering categorized criteria. First, the solution based on the technical criteria, i.e. T1–T5 and E1–E4, is discussed and afterwards the solution based on the economic criteria, i.e. S1 and F1–F3, is analysed. Tables
14 and
15 list the total information contents of all methods considering technical and economic criteria, respectively. Table
16 shows the resultant rankings of technology selection problem based on the two categories.
Table 14
Total information contents considering technical criteria.
Alternatives of waste lubricant oil regenerative technology |
Total information contents |
FAD |
WFAD |
RFAD |
WRFAD |
Acid-clay process |
16 |
8.451 |
18.915 |
8.567 |
Acid-activated clay process |
16 |
8.451 |
18.949 |
8.562 |
Thin/wiped film evaporation |
12 |
6.415 |
13.212 |
6.461 |
Solvent extraction process |
12 |
6.355 |
13.398 |
6.410 |
Hydro-process |
14 |
7.427 |
17.006 |
7.542 |
Table 15
Total information contents considering economic criteria.
Alternatives of waste lubricant oil regenerative technology |
Total information contents |
FAD |
WFAD |
RFAD |
WRFAD |
Acid-clay process |
8 |
4.251 |
8 |
4.251 |
Acid-activated clay process |
8 |
4.251 |
8 |
4.251 |
Thin/wiped film evaporation |
2 |
1.076 |
2.234 |
1.076 |
Solvent extraction process |
6 |
3.176 |
6.454 |
3.181 |
Hydro-process |
6 |
3.176 |
6.690 |
3.183 |
Table 16
Rankings of the candidate technologies considering categorized criteria.
Alternatives of waste lubricant oil regenerative technology |
Rankings based on technical criteria |
Rankings based on economic criteria |
FAD |
WFAD |
RFAD |
WRFAD |
FAD |
WFAD |
RFAD |
WRFAD |
Acid-clay process |
4 |
4 |
4 |
5 |
4 |
4 |
4 |
4 |
Acid-activated clay process |
4 |
4 |
5 |
4 |
4 |
4 |
4 |
4 |
Thin/wiped film evaporation |
1 |
2 |
1 |
2 |
1 |
1 |
1 |
1 |
Solvent extraction process |
1 |
1 |
2 |
1 |
2 |
2 |
2 |
2 |
Hydro-process |
3 |
3 |
3 |
3 |
2 |
2 |
3 |
3 |
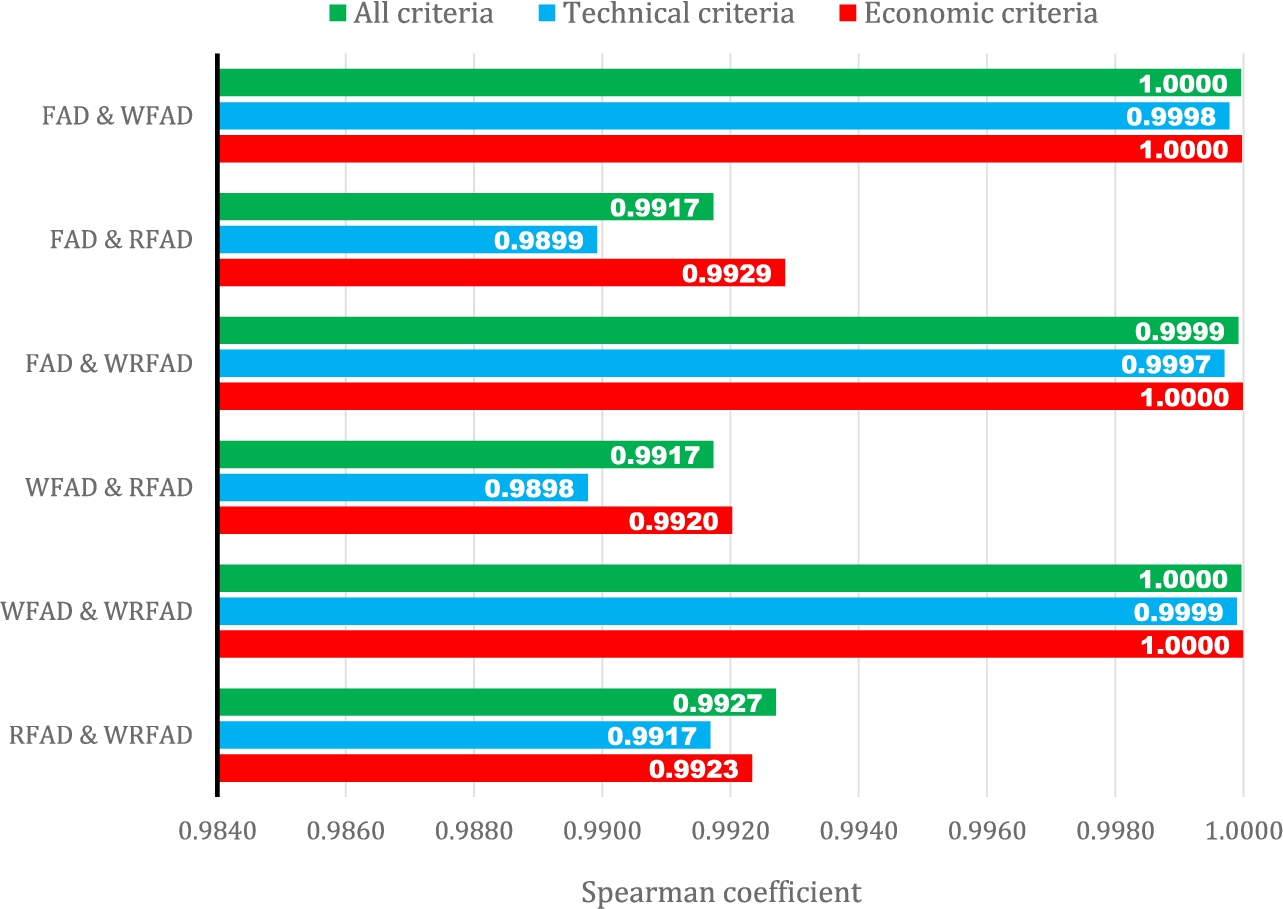
Fig. 6
Correlation between the rankings based on Spearman coefficient considering all and categorized criteria.
The Spearman rank correlation coefficient helps in evaluating similarity of the rankings. The coefficient is a real number in the range of −1 and 1. Spearman coefficient equal to 1 denotes identical rankings and −1 indicates opposite rankings. Fig.
6 illustrates the correlation between ranking lists by utilizing Spearman rank correlation coefficient. Three sets of correlation coefficients are presented in the figure considering all criteria and the two categories. Based on Fig.
6, in the category of technical criteria, the correlation of the methods are lower comparing the category of economic criteria and when all criteria considered for solution. Overall, utilizing all criteria or categorized criteria in the technology selection problem will have different effect on rankings. However, the optimal technology for regenerating used lubricant oils is mostly determined as thin/wiped film evaporation based on Tables
13 and
16.
Thin film evaporation (TFE) or wiped film evaporation (WFE) technology provides short residence time and low pressure drop configuration, allowing continuous and reliable processing of many heat sensitive, viscous, or fouling materials without product degradation. TFE/WFE technology is a high-tech process which is commercially available around the world. Thin film evaporators rapidly separate volatile components employing indirect heat transfer and mechanical turbulence of a flowing product film in supervised circumstances, i.e. using heat jackets and scrapers running along the process and applying high vacuum conditions. Vaporized component or concentrated component (distillate) may be the product depending on the application. This technology has other applications besides recycling of lubricating oils, e.g. tomato pastes. TFE/WFE technology selected from the decision-making process has some advantages compared to other alternatives, e.g. environmental and safety risks of the technology is very low, also the marginal profit of process is higher than other technologies.
5 Conclusion
In today’s dynamic and competitive environment of production companies, selecting the best available technology is substantial. In high-tech production lines, selecting the optimal technology is a tough task which may require given experiments and special experiences. The information in the technology selection problems related to such environments because of existence of uncertainties may entail risks. Selecting the optimal lubricant oil regenerative technology considering sustainability, environmental issues, and stakeholders’ satisfaction may cause unexpected scenarios and conditions which increase the possibility of risks. Because of the current environmental concerns in the 21st century, there is a heavy criticism against the usage of fossil fuels and hydrocarbon compositions. Therefore, decision-making about the selection of appropriate waste lubricant oil regenerative technology is a weighty responsibility which is discussed in the current research.
The current study presented a new practical industrial application for the RFAD method. Considering risk factors in the proposed algorithm, a practical technology selection example about used lubricant oil regenerative technologies was considered. In the present paper, risk factors were first identified and a comprehensive description of general and specific risk factors in waste lubricant oil regenerative technologies was provided. Second, the extended version of the RFAD approach, i.e. WRFAD, with the integrated Shannon entropy significance coefficients was utilized to provide a risk-based technology selection algorithm that is applicable for high-tech production plants. Third, two attitudes for solving the problem were adopted, a technology selection based on every criterion, and a solution by dividing all criteria into two major categories. Finally, the correlations between the rankings were examined by applying Spearman rank correlation coefficients.
The decision matrix of the case study contains five potential technologies which are currently exploited in modern regenerated lubricant oils production lines. The system ranges of the example are triangular fuzzy ratings determined based on the experts’ comments. Information contents were calculated employing AD principles.
Suggestions for future developments of this study may be as follows. First, input data of the AD approach can be extended for the cases in which the data of the problem has different mathematical forms such as the extensions of fuzzy sets, e.g. L-fuzzy sets, flou sets, fuzzy multi-sets, and bipolar fuzzy sets. An interesting form of uncertain decision making is linguistic decision analysis. The linguistic assessment is more flexible and user friendly to represent preferences of decision makers (Cabrerizo
et al.,
2013,
2014). Second, significance coefficients of criteria may be achieved using various techniques. In the current study, subjective significance coefficients were considered and objective significance coefficients were determined based on Shannon entropy. Subjective significance coefficients may be computed applying various methods like the ANP and AHP. Third, the introduced technology selection algorithm can be employed in other industrial activities in which the importance of risk factors are high like municipal solid waste management and polychlorinated biphenyl (PCB) treatment. Fourth, in the current study, many general and specified risk factors are identified and described for the practical case. However, some unseen risk factors may exist that can be recognized based on systematic analyses.