1 Introduction
Selection of the most suitable site for a residential area is one of the conditions determining the quality of living in urban cities. Residential construction site selection problem requires operational, environmental, social, and economic criteria to be considered in the assessment process. These criteria may be intangible, tangible and conflicting with each other. The assessment process is generally realized under vague and imprecise environment, which justifies the usage of the fuzzy set theory.
Residential construction site selection problem can be solved by a multi-criterion decision-making (MCDM) method. MCDM methods help decision-makers to subjectively evaluate the performance of alternatives with respect to the predetermined criteria (Zavadskas
et al.,
2004,
2014). In the literature, there are many MCDM methods such as Analytic Hierarchy Process (AHP) (Saaty,
1980), Technique for Order of Preference by Similarity to Ideal Solution (TOPSIS) (Yoon and Hwang,
1981), ELimination Et Choix Traduisant la REalité (ELECTRE) (Roy,
1991), Analytic Network Process (ANP) (Saaty,
1996), Evaluation Based on Distance from Average Solution (EDAS) (Keshavarz Ghorabaee
et al.,
2015), and Combinative Distance-Based Assessment (CODAS) (Keshavarz Ghorabaee
et al.,
2016). These methods are constructed not only to handle the environmental, human, and social aspects of the problem but also to correctly capture the uncertainties in these aspects. Since residential construction site selection problems involve many uncertainties in terms of vagueness and impreciseness, the best way is to use fuzzy extensions of these MCDM methods.
Fuzzy sets theory was introduced by Zadeh to capture the uncertainties in human thoughts through the degree of memberships of the elements in a set (Zadeh,
1965). In order to increase the capability of handling vagueness and impreciseness in the problems, ordinary fuzzy sets have been extended to many types. Type-n fuzzy sets were developed by Zadeh to reduce the uncertainty of the membership functions in the ordinary fuzzy sets (Zadeh,
1975). Interval-valued fuzzy sets were introduced independently by Zadeh (
1975), Grattan-Guiness (
1975), Sambuc (
1975), Jahn (
1975). Intuitionistic fuzzy sets were introduced by Atanassov to show how the hesitancy degree of a decision maker can be handled (Atanassov,
1986). Smarandache developed neutrosophic sets for demonstrating the differences between relativity and absoluteness in the decision makers’ preferences (Smarandache,
2005). Hesitant fuzzy sets (HFSs) initially described by Torra (
2010) are the extensions of ordinary fuzzy sets where a set of values are possible for the membership of a single element (Torra,
2010). Classical MCDM methods have been extended to their fuzzy versions using these types of fuzzy sets: intuitionistic fuzzy EDAS (Kahraman
et al.,
2017), ordinary fuzzy CODAS (Keshavarz Ghorabaee
et al.,
2017), type-2 fuzzy AHP (Kahraman
et al.,
2014), hesitant TOPSIS (Xu and Zhang,
2013), neutrosophic ELECTRE III. In the literature, setting linguistic scale is essentially realized in two ways: the studies using a constant linguistic scale as in Kwong and Bai (
2003), Kulak and Kahraman (
2005) and the studies using tools such as mathematical programming or statistical modelling to determine the intervals corresponding to the linguistic terms as reviewing in Liao
et al. (
2018) and applied in Cabrerizo
et al. (
2017). Since our paper falls in the studies using a constant linguistic scale, we developed linguistic scales corresponding to fuzzy numbers for our paper.
CODAS is a distance based MCDM method proposed by Keshavarz Ghorabaee
et al. (
2016). In this method, the overall performance of an alternative is measured by the Euclidean and Taxicab distances from the negative-ideal point. The CODAS uses the Euclidean distance as the primary measure of assessment. If the Euclidean distances of two alternatives are very close to each other, the Hamming distance is used to compare them. The degree of closeness of Euclidean distances is set by a threshold parameter (Keshavarz Ghorabaee
et al.,
2016). It is quite a new method in the literature but thanks to its advantages it is expected to be used more in the future.
In this paper, a novel hesitant fuzzy CODAS method is developed and applied to the selection of a residential construction site project. The originality of this paper can be explained by three items. Firstly, we develop a novel fuzzy CODAS method and apply it to a residential construction site selection problem. Secondly, in the proposed method, the weights of the criteria are obtained by hesitant fuzzy AHP method which makes our approach an integrated methodology. Finally, for validating the proposed method, we compare our results with the results of ordinary fuzzy CODAS method. An explanatory sensitivity analysis is also performed to demonstrate the stability of the ranking results of the hesitant fuzzy CODAS method.
The rest of the paper is organized as follows: In Section
2, a literature review on construction site selection problems is given. In Section
3, the steps of ordinary fuzzy CODAS method are presented. In Section
4, the proposed methodology is clarified with its details. In Section
5, the proposed method is applied to a residential construction site selection problem. The paper ends with conclusions and suggestions for further research.
2 Literature Review: Construction Site Selection Problems
Numerous MCDM models have been developed for evaluating construction site location alternatives with respect to the predetermined criteria. We have analysed the studies that can be beneficial for our application and have presented a general evaluation of them. In these studies, MCDM methods are mainly applied for obtaining the solutions of the site selection problems in many different areas.
Cheng
et al. (
2003) studied MCDM methods to support selection of an optimal landfill site and a waste-flow-allocation pattern. Zavadskas
et al. (
2004) applied ELECTRE III method for the selection of the best commercial construction project. It is emphasized that MCDM methods are quite suitable for the evaluation and decision-making assessments for construction projects. Dey and Ramcharan (
2008) applied AHP method for the site selection process of expansion on limestone quarry operations to support cement production in Barbados. The results show that AHP is an effective method of decision-making and can consider both objective and subjective factors. Turskis
et al. (
2012) studied the determination of the best construction site alternative for non-hazardous waste incineration plant by using ARAS-F and AHP methods. It can be deducted based on the results that performing more precise assessments is possible with fuzzy sets theory. Balali
et al. (
2012) applied a new algorithm combining ELECTRE III and Preference Ranking Organization Method for Enrich Evaluation II (PROMETHEE II) for decision-making in the construction management processes. Eskandari
et al. (
2012) presented a study of landfill site selection problem by integrating geographic information systems (GIS) and AHP method. Hasanzadeh
et al. (
2013) performed an application of AHP for prioritizing the environmental criteria of coastal oil jetties. The results of the study indicate that ANP findings have a high efficiency for weighting the importance degrees of criteria for environmental construction. Bagocius
et al. (
2014) presented a study about the selection of the most appropriate location for a liquefied natural gas terminal based on the results of different MCDM methods. Results of the study indicate that outcomes of SAW, TOPSIS, and COPRAS methods are consistent and give similar consequences. Zavadskas
et al. (
2015) applied Weighted Aggregated Sum Product Assessment (WASPAS) method with single-valued neutrosophic sets. The results of the study indicate that applied neutrosophic MCDM method is quite efficient and meets the requirements for the evaluation of intangible factors of the problem. Mousavi
et al. (
2015) investigated the suitability of the Kish Island coastal areas for the establishment of artificial corals reefs using spatial MCDM tool. Results of the study demonstrated that weighted linear combination method should be used for the identification of alternatives and AHP should be used for the prioritization of alternatives. Chaudhary
et al. (
2016) studied fire station suitability zonation mapping of Kathmandu City and determined the best alternative using GIS and AHP methods. Since the results reveal that 13.46% of the considered area is highly suitable for fire station location, zonation map is trustworthy and can be used for the construction of fire stations. Bahrani
et al. (
2016) presented a study on landfill site selection by using fuzzy GIS and ordinary AHP. The authors demonstrated that fuzzy functions for landfill site selection were way better than crisp ones for GIS. Bansal
et al. (
2017) presented a fuzzy decision approach which is a combination of fuzzy synthetic evaluation method and analytic hierarchy process for the selection of most suitable construction method of green buildings. The results show that the proposed model can be an analytical tool to evaluate the applicability of prefabricated or on-site construction methods. Chen
et al. (
2018) studied another construction site location selection problem by applying EDAS and WASPAS methods. They conducted Monte Carlo simulation to check the sensitivity in changes of the criterion weights.
In this paper, we propose a novel hesitant fuzzy CODAS method which provides flexibility to the definition of membership function and to the measurement of distances from negative-ideal solution. In hesitant fuzzy sets, the difficulty in establishing the membership degrees does not arise from a margin of error or a specified possibility distribution of the possible values but arises from our hesitation among a few different values (Zhang,
2013). Thus, the proposed model can make a comprehensive evaluation in terms of both fuzziness and distance measurement, allowing a more accurate representation of knowledge.
5 Application
A council consisting of Metropolitan Municipality Directors, Housing Development Administration and Contractors’ representatives would like to determine the location of a residential which has 10,000 residences to be built in the city of Istanbul. The council determined 8 alternative construction sites whose locations are indicated in Fig.
2. The evaluation factors consist of 4 main criteria and 14 sub criteria. The aim is to find the best alternative for the residential construction site based on the pre-determined criteria with respect to the council’s opinions. The weights of the criteria are obtained by hesitant fuzzy AHP (Tuysuz and Simsek,
2017). These weights are used in the proposed hesitant fuzzy CODAS to obtain the weighted normalized decision matrix. The results of the integrated methodology are verified with the sensitivity analysis. A comparative analysis is also conducted to show the validation of the proposed method.
5.1 Problem Definition
The contractors agreed to use a scientific method to determine the most appropriate site from the alternate locations in order to obtain the approval of the residential. They formed an academicians’ committee composed of 4 people that would carry out the application. Since the problem has too many criteria and alternatives, the committee has decided to use some MCDM methods including our integrated methodology for the solution of this problem. In our integrated methodology, the weights of the criteria are determined by hesitant fuzzy AHP and then, the proposed hesitant fuzzy CODAS method is applied to obtain the best residential construction site. The determined criteria for implementation are given in Table
2.
Table 2
Determined criteria for the application.
Social Criteria |
Attractiveness of land |
Economic Criteria |
Price |
Population characteristics |
Infrastructure cost |
Distance from historical sites |
Construction cost |
Distance from other residential areas |
Slope of the land |
Environmental Criteria |
Forestland |
Technical Criteria |
Distance from waste production centers |
Agricultural land |
Distance from high-standard roads |
Human and animal habitats |
Distance from industrial areas |
The hierarchy involving the specified criteria and alternatives is given in Fig.
3.
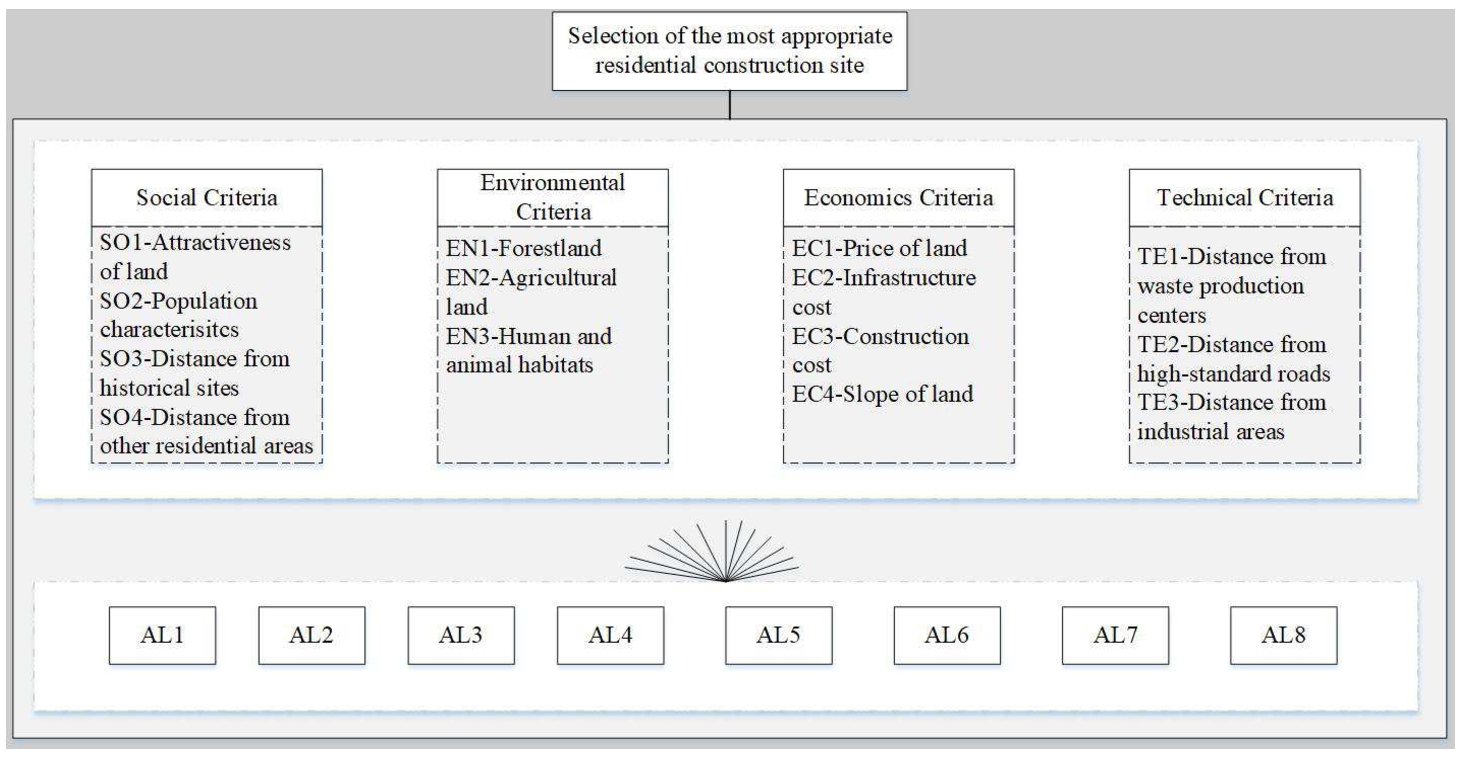
Fig. 3
Hierarchy of the application.
5.2 Solution of Application
As the first step of our proposed hesitant fuzzy CODAS method, the initial decision matrix involving linguistic assessments is constructed in Table
3. In this table, the committee can assign different linguistic evaluations for each criterion. The number of these evaluations may change from one to four since the hesitant fuzzy approach requires it. The hyphens in the table indicate that a member of the committee did not prefer making an evaluation for the related alternative with respect to the considered criterion.
Table 3
Decision matrix with linguistic terms.
|
SO1 |
SO2 |
SO3 |
SO4 |
EN1 |
EN2 |
EN3 |
AL1 |
MG |
VP |
– |
VG |
MG |
– |
F |
MG |
MG |
G |
MG |
MG |
VG |
– |
MG |
F |
F |
G |
F |
F |
F |
P |
– |
VP |
P |
MP |
VP |
VP |
AL2 |
– |
MP |
VP |
VP |
VG |
MP |
– |
MG |
G |
MP |
F |
VG |
F |
G |
G |
MP |
– |
F |
– |
MP |
UI |
F |
VG |
G |
F |
MG |
G |
MP |
AL3 |
P |
VG |
MP |
F |
F |
MG |
G |
– |
VG |
MP |
– |
P |
– |
MP |
VP |
– |
P |
MG |
– |
G |
– |
VP |
MP |
MG |
G |
MP |
MP |
F |
AL4 |
P |
VG |
– |
VP |
MG |
– |
F |
VG |
VG |
F |
MG |
VG |
– |
G |
VG |
MG |
VP |
P |
G |
G |
MG |
– |
VG |
F |
P |
VG |
MP |
G |
AL5 |
VG |
MG |
SP |
VG |
G |
F |
MP |
SP |
G |
VP |
F |
P |
MG |
– |
VG |
VP |
MP |
VP |
G |
MG |
MP |
VG |
MG |
MP |
– |
P |
G |
F |
AL6 |
VG |
VP |
G |
P |
MG |
F |
G |
MP |
MG |
F |
MP |
P |
– |
G |
G |
MP |
VG |
F |
VG |
MG |
MG |
VG |
MP |
MP |
– |
– |
VP |
MG |
AL7 |
MG |
P |
F |
VG |
F |
VG |
MP |
G |
– |
G |
G |
P |
VG |
VP |
MG |
MG |
F |
G |
F |
MP |
P |
MP |
MG |
F |
VP |
VP |
G |
P |
AL8 |
F |
VP |
F |
– |
F |
VP |
– |
VP |
P |
P |
G |
VG |
MG |
VG |
F |
VP |
MG |
– |
P |
MP |
VG |
– |
F |
G |
P |
P |
G |
G |
|
EC1 |
EC2 |
EC3 |
EC4 |
TE1 |
TE2 |
TE3 |
AL1 |
P |
VP |
– |
F |
MG |
– |
G |
F |
P |
G |
MP |
P |
P |
VG |
MG |
P |
G |
VG |
VP |
VG |
VG |
– |
– |
MG |
G |
VP |
MG |
UI |
AL2 |
MP |
MG |
P |
MG |
G |
G |
MG |
VG |
MP |
MP |
F |
VG |
MG |
MP |
G |
MP |
VP |
VP |
MG |
P |
P |
VG |
P |
– |
– |
VG |
G |
– |
AL3 |
G |
G |
MP |
VG |
F |
G |
MP |
F |
VP |
G |
G |
MG |
VG |
P |
VG |
P |
MG |
P |
VP |
MG |
MG |
VP |
F |
VP |
MP |
G |
– |
VP |
AL4 |
MP |
P |
– |
G |
VP |
P |
VG |
MG |
F |
– |
F |
MG |
MP |
– |
P |
G |
VP |
VP |
MG |
MP |
MP |
VP |
MP |
F |
F |
P |
P |
MP |
AL5 |
P |
MP |
– |
P |
MP |
MG |
– |
G |
F |
F |
F |
MG |
F |
MP |
P |
F |
G |
MG |
VG |
G |
F |
MG |
G |
F |
MP |
MP |
VP |
G |
AL6 |
VP |
VG |
F |
MP |
MP |
G |
F |
– |
VP |
– |
F |
– |
VG |
P |
P |
– |
MG |
G |
MG |
F |
VG |
MG |
VP |
MP |
VP |
F |
MP |
G |
AL7 |
VG |
MG |
F |
VG |
P |
MG |
– |
MP |
P |
VG |
F |
P |
MP |
– |
F |
P |
VG |
P |
– |
P |
MP |
VG |
VG |
P |
G |
MP |
G |
MP |
AL8 |
P |
G |
– |
– |
VG |
VP |
F |
G |
MG |
MP |
F |
VP |
MG |
VP |
VG |
P |
VP |
G |
– |
VP |
VG |
F |
MP |
G |
UI |
VP |
VG |
G |
Secondly, the initial decision matrix is converted to the decision matrix with corresponding numerical membership degrees. The decision matrix is constructed in Table
4. After this point of the method, we partially present the normalized decision matrix (Table
5) and weighted normalized decision matrix (Table
6) because of the space constraints.
Table 4
Decision matrix with membership degrees.
|
BENEFIT |
BENEFIT |
BENEFIT |
BENEFIT |
COST |
COST |
COST |
|
0.0464 |
0.0680 |
0.0783 |
0.0227 |
0.0702 |
0.1140 |
0.1316 |
|
SO1 |
SO2 |
SO3 |
SO4 |
EN1 |
EN2 |
EN3 |
|
MS1 |
MS2 |
MS3 |
MS4 |
MS1 |
MS2 |
MS3 |
MS4 |
MS1 |
MS2 |
MS3 |
MS4 |
MS1 |
MS2 |
MS3 |
MS4 |
MS1 |
MS2 |
MS3 |
MS4 |
MS1 |
MS2 |
MS3 |
MS4 |
MS1 |
MS2 |
MS3 |
MS4 |
AL1 |
[6.3, 8.1] |
[4.5, 6.3] |
[0.9, 2.7] |
[0.9, 2.7] |
[4.5, 6.3] |
[4.5, 6.3] |
[3.6, 5.4] |
[3.6, 5.4] |
[5.4, 7.2] |
[4.5, 6.3] |
[4.5, 6.3] |
[4.5, 6.3] |
[6.3, 8.1] |
[4.5, 6.3] |
[3.6, 5.4] |
[3.6, 5.4] |
[3.6, 5.4] |
[3.6, 5.4] |
[3.6, 5.4] |
[3.6, 5.4] |
[3.6, 5.4] |
[1.8, 3.6] |
[0.9, 2.7] |
[0.9, 2.7] |
[2.7, 4.5] |
[1.8, 3.6] |
[0.9, 2.7] |
[0.9, 2.7] |
AL2 |
[2.7, 4.5] |
[0.9, 2.7] |
[0.9, 2.7] |
[0.9, 2.7] |
[6.3, 8.1] |
[4.5, 6.3] |
[2.7, 4.5] |
[2.7, 4.5] |
[6.3, 8.1] |
[5.4, 7.2] |
[3.6, 5.4] |
[2.7, 4.5] |
[5.4, 7.2] |
[5.4, 7.2] |
[3.6, 5.4] |
[2.7, 4.5] |
[2.7, 4.5] |
[2.7, 4.5] |
[2.7, 4.5] |
[2.7, 4.5] |
[6.3, 8.1] |
[5.4, 7.2] |
[3.6, 5.4] |
[0.1, 1.8] |
[5.4, 7.2] |
[4.5, 6.3] |
[3.6, 5.4] |
[2.7, 4.5] |
AL3 |
[6.3, 8.1] |
[3.6, 5.4] |
[2.7, 4.5] |
[1.8, 3.6] |
[5.4, 7.2] |
[4.5, 6.3] |
[3.6, 5.4] |
[3.6, 5.4] |
[6.3, 8.1] |
[2.7, 4.5] |
[1.8, 3.6] |
[1.8, 3.6] |
[2.7, 4.5] |
[0.9, 2.7] |
[0.9, 2.7] |
[0.9, 2.7] |
[1.8, 6.3] |
[4.5, 6.3] |
[1.8, 3.6] |
[1.8, 3.6] |
[4.5, 6.3] |
[2.7, 4.5] |
[0.9, 2.7] |
[0.9, 2.7] |
[5.4, 7.2] |
[3.6, 5.4] |
[2.7, 4.5] |
[2.7, 4.5] |
AL4 |
[6.3, 8.1] |
[1.8, 3.6] |
[0.9, 2.7] |
[0.9, 2.7] |
[6.3, 8.1] |
[4.5, 6.3] |
[3.6, 5.4] |
[3.6, 5.4] |
[6.3, 8.1] |
[6.3, 8.1] |
[4.5, 6.3] |
[3.6, 5.4] |
[6.3, 8.1] |
[5.4, 7.2] |
[4.5, 6.3] |
[4.5, 6.3] |
[4.5, 7.2] |
[5.4, 7.2] |
[1.8, 3.6] |
[0.9, 2.7] |
[6.3, 8.1] |
[4.5, 6.3] |
[3.6, 5.4] |
[3.6, 5.4] |
[6.3, 8.1] |
[5.4, 7.2] |
[2.7, 4.5] |
[1.8, 3.6] |
AL5 |
[7.2, 9] |
[6.3, 8.1] |
[6.3, 8.1] |
[4.5, 6.3] |
[7.2, 9] |
[5.4, 7.2] |
[3.6, 5.4] |
[2.7, 4.5] |
[5.4, 7.2] |
[3.6, 5.4] |
[1.8, 3.6] |
[0.9, 2.7] |
[6.3, 8.1] |
[4.5, 6.3] |
[0.9, 2.7] |
[0.9, 2.7] |
[2.7, 6.3] |
[4.5, 6.3] |
[2.7, 4.5] |
[0.9, 2.7] |
[6.3, 8.1] |
[4.5, 6.3] |
[2.7, 4.5] |
[2.7, 4.5] |
[5.4, 7.2] |
[3.6, 5.4] |
[1.8, 3.6] |
[1.8, 3.6] |
AL6 |
[6.3, 8.1] |
[5.4, 6.3] |
[1.8, 3.6] |
[0.9, 2.7] |
[5.4, 7.2] |
[4.5, 6.3] |
[3.6, 4.5] |
[2.7, 4.5] |
[4.5, 6.3] |
[3.6, 5.4] |
[2.7, 4.5] |
[1.8, 3.6] |
[5.4, 7.2] |
[5.4, 7.2] |
[2.7, 4.5] |
[2.7, 4.5] |
[6.3, 8.1] |
[6.3, 8.1] |
[4.6, 6.3] |
[3.6, 5.4] |
[6.3, 8.1] |
[4.5, 6.3] |
[2.7, 4.5] |
[2.7, 4.5] |
[4.5, 6.3] |
[0.9, 2.7] |
[0.9, 2.7] |
[0.9, 2.7] |
AL7 |
[6.3, 8.1] |
[5.4, 6.3] |
[4.5, 6.3] |
[1.8, 3.6] |
[6.3, 8.1] |
[5.4, 7.2] |
[4.5, 5.4] |
[3.6, 5.4] |
[5.4, 7.2] |
[5.4, 7.2] |
[1.8, 3.6] |
[1.8, 3.6] |
[6.3, 8.1] |
[4.5, 6.3] |
[4.5, 6.3] |
[0.9, 2.7] |
[3.6, 5.4] |
[3.6, 5.4] |
[3.6, 5.4] |
[2.7, 4.5] |
[4.5, 6.3] |
[3.6, 5.4] |
[2.7, 4.5] |
[1.8, 3.6] |
[5.4, 7.2] |
[1.8, 3.6] |
[0.9, 2.7] |
[0.9, 2.7] |
AL8 |
[3.6, 5.4] |
[3.6, 5.4] |
[0.9, 2.7] |
[0.9, 2.7] |
[3.6, 5.4] |
[0.9, 2.7] |
[0.9, 2.7] |
[0.9, 2.7] |
[6.3, 8.1] |
[5.4, 7.2] |
[1.8, 3.6] |
[1.8, 3.6] |
[6.3, 8.1] |
[4.5, 6.3] |
[3.6, 5.4] |
[0.9, 2.7] |
[2.7, 3.6] |
[2.7, 4.5] |
[1.8, 3.6] |
[1.8, 3.6] |
[6.3, 8.1] |
[5.4, 7.2] |
[3.6, 5.4] |
[3.6, 5.4] |
[5.4, 7.2] |
[5.4, 7.2] |
[1.8, 3.6] |
[1.8, 3.6] |
|
COST |
COST |
COST |
COST |
BENEFIT |
COST |
COST |
|
0.0808 |
0.1313 |
0.1515 |
0.0227 |
0.0332 |
0.0383 |
0.1313 |
|
EC1 |
EC2 |
EC3 |
EC4 |
TE1 |
TE2 |
TE3 |
|
MS1 |
MS2 |
MS3 |
MS4 |
MS1 |
MS2 |
MS3 |
MS4 |
MS1 |
MS2 |
MS3 |
MS4 |
MS1 |
MS2 |
MS3 |
MS4 |
MS1 |
MS2 |
MS3 |
MS4 |
MS1 |
MS2 |
MS3 |
MS4 |
MS1 |
MS2 |
MS3 |
MS4 |
AL1 |
[3.6, 5.4] |
[1.8, 3.6] |
[0.9, 2.7] |
[0.9, 2.7] |
[5.4, 7.2] |
[5.4, 5.4] |
[3.6, 4.5] |
[3.6, 4.5] |
[5.4, 7.2] |
[2.7, 4.5] |
[1.8, 3.6] |
[1.8, 3.6] |
[6.3, 8.1] |
[4.5, 6.3] |
[1.8, 3.6] |
[1.8, 3.6] |
[6.3, 8.1] |
[6.3, 8.1] |
[5.4, 7.2] |
[0.9, 2.7] |
[6.3, 8.1] |
[4.5, 6.3] |
[4.5, 6.3] |
[4.5, 6.3] |
[5.4, 7.2] |
[4.5, 6.3] |
[0.9, 2.7] |
[0.1, 1.8] |
AL2 |
[4.5, 6.3] |
[4.5, 6.3] |
[2.7, 4.5] |
[1.8, 3.6] |
[6.3, 8.1] |
[7, 7.2] |
[5.4, 8] |
[6, 7] |
[6.3, 8.1] |
[3.6, 5.4] |
[2.7, 4.5] |
[2.7, 4.5] |
[5.4, 7.2] |
[4.5, 6.3] |
[2.7, 4.5] |
[2.7, 4.5] |
[4.5, 6.3] |
[2.7, 4.5] |
[1.8, 3.6] |
[0.9, 2.7] |
[6.3, 8.1] |
[1.8, 3.6] |
[1.8, 3.6] |
[1.8, 3.6] |
[6.3, 8.1] |
[5.4, 7.2] |
[5.4, 7.2] |
[5.4, 7.2] |
AL3 |
[6.3, 8.1] |
[5.4, 7.2] |
[5.4, 7.2] |
[2.7, 4.5] |
[5.4, 7.2] |
[3.6, 5.4] |
[3.6, 5.4] |
[2.7, 4.5] |
[5.4, 7.2] |
[5.4, 7.2] |
[4.5, 6.3] |
[0.9, 2.7] |
[6.3, 8.1] |
[6.3, 8.1] |
[1.8, 3.6] |
[1.8, 3.6] |
[4.5, 6.3] |
[4.5, 6.3] |
[1.8, 3.6] |
[0.9, 2.7] |
[4.5, 6.3] |
[3.6, 5.4] |
[0.9, 2.7] |
[0.9, 2.7] |
[5.4, 7.2] |
[2.7, 4.5] |
[0.9, 2.7] |
[0.9, 2.7] |
AL4 |
[5.4, 7.2] |
[3.6, 4.5] |
[1.8, 2.7] |
[1.8, 2.7] |
[6.3, 8.1] |
[4.5, 6.3] |
[1.8, 3.6] |
[0.9, 2.7] |
[4.5, 6.3] |
[3.6, 5.4] |
[3.6, 5.4] |
[3.6, 5.4] |
[5.4, 7.2] |
[2.7, 4.5] |
[1.8, 3.6] |
[1.8, 3.6] |
[4.5, 6.3] |
[2.7, 4.5] |
[2.7, 4.5] |
[0.9, 2.7] |
[3.6, 5.4] |
[2.7, 4.5] |
[2.7, 4.5] |
[0.9, 2.7] |
[3.6, 5.4] |
[2.7, 4.5] |
[1.8, 3.6] |
[1.8, 3.6] |
AL5 |
[2.7, 4.5] |
[1.8, 3.6] |
[1.8, 3.6] |
[1.8, 3.6] |
[5.4, 7.2] |
[4.5, 6.3] |
[2.7, 4.5] |
[2.7, 4.5] |
[4.5, 6.3] |
[3.6, 5.4] |
[3.6, 5.4] |
[3.6, 5.4] |
[3.6, 5.4] |
[3.6, 5.4] |
[2.7, 4.5] |
[1.8, 3.6] |
[6.3, 8.1] |
[5.4, 7.2] |
[5.4, 7.2] |
[4.5, 6.3] |
[5.4, 7.2] |
[4.5, 6.3] |
[3.6, 5.4] |
[3.6, 5.4] |
[5.4, 7.2] |
[2.7, 4.5] |
[2.7, 4.5] |
[1.8, 3.6] |
AL6 |
[6.3, 8.1] |
[3.6, 5.4] |
[2.7, 4.5] |
[0.9, 2.7] |
[5.4, 7.2] |
[3.6, 5.4] |
[2.7, 4.5] |
[2.7, 4.5] |
[3.6, 5.4] |
[0.9, 2.7] |
[0.9, 2.7] |
[0.9, 2.7] |
[6.3, 8.1] |
[1.8, 3.6] |
[1.8, 3.6] |
[1.8, 3] |
[5.4, 7.2] |
[4.5, 6.3] |
[4.5, 6.3] |
[3.6, 5.4] |
[6.3, 8.1] |
[4.5, 6.3] |
[2.7, 4.5] |
[0.9, 2.7] |
[5.4, 7.2] |
[3.6, 5.4] |
[2.7, 4.5] |
[0.9, 2.7] |
AL7 |
[6.3, 8.1] |
[6.3, 8.1] |
[4.5, 6.3] |
[3.6, 5.4] |
[4.5, 6.3] |
[2.7, 4.5] |
[1.8, 3.6] |
[1.8, 3.6] |
[6.3, 8.1] |
[3.6, 5.4] |
[1.8, 3.6] |
[1.8, 3.6] |
[3.6, 5.4] |
[2.7, 4.5] |
[1.8, 3.6] |
[1.8, 3.6] |
[6.3, 8.1] |
[1.8, 3.6] |
[1.8, 3.6] |
[1.8, 3.6] |
[6.3, 8.1] |
[6.3, 8.1] |
[2.7, 4.5] |
[1.8, 3.6] |
[5.4, 7.2] |
[5.4, 7.2] |
[2.7, 4.5] |
[2.7, 4.5] |
AL8 |
[5.4, 7.2] |
[1.8, 3.6] |
[1.8, 3.6] |
[1.8, 3.6] |
[6.3, 8.1] |
[5.4, 7.2] |
[3.6, 5.4] |
[0.9, 2.7] |
[4.5, 6.3] |
[3.6, 5.4] |
[2.7, 3.6] |
[0.9, 2.7] |
[6.3, 8.1] |
[4.5, 6.3] |
[1.8, 3.6] |
[0.9, 2.7] |
[5.4, 7.2] |
[0.9, 2.7] |
[0.9, 2.7] |
[0.9, 2.7] |
[6.3, 8.1] |
[5.4, 7.2] |
[3.6, 5.4] |
[2.7, 4.5] |
[6.3, 8.1] |
[5.4, 7.2] |
[0.9, 2.7] |
[0.1, 1.8] |
Table 5
Normalized decision matrix.
Type |
BENEFIT |
|
COST |
Weight |
0.0464 |
|
0.1313 |
Criteria |
SO1 |
|
TE3 |
Membership |
MS1 |
MS2 |
MS3 |
MS4 |
|
MS1 |
MS2 |
MS3 |
MS4 |
AL1 |
[0.7, 0.9] |
[0.56, 0.78] |
[0.11, 0.33] |
[0.14, 0.43] |
|
[0.5, 0.67] |
[0.43, 0.6] |
[0.33, 1] |
[0.06, 1] |
AL2 |
[0.3, 0.5] |
[0.11, 0.33] |
[0.11, 0.33] |
[0.14, 0.43] |
|
[0.44, 0.57] |
[0.38, 0.5] |
[0.13, 0.17] |
[0.01, 0.02] |
AL3 |
[0.7, 0.9] |
[0.44, 0.67] |
[0.33, 0.56] |
[0.29, 0.57] |
|
[0.5, 0.67] |
[0.6, 1] |
[0.33, 1] |
[0.04, 0.11] |
AL4 |
[0.7, 0.9] |
[0.22, 0.44] |
[0.11, 0.33] |
[0.14, 0.43] |
|
[0.67, 1] |
[0.6, 1] |
[0.25, 0.5] |
[0.03, 0.06] |
AL5 |
[0.8, 1] |
[0.78, 1] |
[0.78, 1] |
[0.71, 1] |
|
[0.5, 0.67] |
[0.6, 1] |
[0.2, 0.33] |
[0.03, 0.06] |
AL6 |
[0.7, 0.9] |
[0.67, 0.78] |
[0.22, 0.44] |
[0.14, 0.43] |
|
[0.5, 0.67] |
[0.5, 0.75] |
[0.2, 0.33] |
[0.04, 0.11] |
AL7 |
[0.7, 0.9] |
[0.67, 0.78] |
[0.56, 0.78] |
[0.29, 0.57] |
|
[0.5, 0.67] |
[0.38, 0.5] |
[0.2, 0.33] |
[0.02, 0.04] |
AL8 |
[0.4, 0.6] |
[0.44, 0.67] |
[0.11, 0.33] |
[0.14, 0.43] |
|
[0.44, 0.57] |
[0.38, 0.5] |
[0.33, 1] |
[0.06, 1] |
Table 6
Weightednormalized decision matrix.
Type |
BENEFIT |
COST |
Weight |
0.0464 |
0.1313 |
Criteria |
SO1 |
TE3 |
Membership |
MS1 |
MS2 |
MS3 |
MS4 |
MS1 |
MS2 |
MS3 |
MS4 |
AL1 |
[0.054, 0.101] |
[0.037, 0.067] |
[0.005, 0.019] |
[0.007, 0.026] |
[0.052, 1] |
[0.052, 1] |
[0.054, 0.106] |
[0.054, 1] |
AL2 |
[0.016, 0.032] |
[0.005, 0.019] |
[0.005, 0.019] |
[0.007, 0.026] |
[0.024, 0.037] |
[0.029, 0.052] |
[0.044, 0.071] |
[0.027, 0.04] |
AL3 |
[0.054, 0.101] |
[0.027, 0.05] |
[0.019, 0.037] |
[0.015, 0.039] |
[0.029, 0.052] |
[0.029, 0.052] |
[0.032, 0.044] |
[0.023, 0.032] |
AL4 |
[0.054, 0.101] |
[0.012, 0.027] |
[0.005, 0.019] |
[0.007, 0.026] |
[0.029, 0.052] |
[0.037, 0.087] |
[0.037, 0.054] |
[0.04, 0.054] |
AL5 |
[0.072, 1] |
[0.067, 1] |
[0.067, 1] |
[0.056, 1] |
[0.037, 0.087] |
[0.037, 0.087] |
[0.071, 1] |
[0.054, 1] |
AL6 |
[0.054, 0.101] |
[0.05, 0.067] |
[0.012, 0.027] |
[0.007, 0.026] |
[0.052, 1] |
[0.052, 1] |
[0.032, 0.044] |
[0.032, 0.054] |
AL7 |
[0.054, 0.101] |
[0.05, 0.067] |
[0.037, 0.067] |
[0.015, 0.039] |
[0.052, 1] |
[0.052, 1] |
[0.032, 0.044] |
[0.02, 0.027] |
AL8 |
[0.023, 0.042] |
[0.027, 0.05] |
[0.005, 0.019] |
[0.007, 0.026] |
[0.037, 0.087] |
[0.037, 0.087] |
[0.037, 0.054] |
[0.054, 1] |
As the next step, we calculate the negative-ideal solution by using Eq. (
35). The negative solution is found as
$(\langle [0.016,0.032],[0.005,0.019],[0.005,0.019],[0.007,0.026]\rangle $,
$\langle [0.034,0.06],[0.009,0.031],[0.012,0.046],[0.012,0.046]\rangle $,
$\langle [0.062,0.111],[0.031,0.062],[0.026,0.064],[0.012,0.043]\rangle $,
$\langle [0.009,0.018],[0.003,0.011],[0.003,0.013],[0.003,0.013]\rangle $,
$\langle [0.023,0.023],[0.028,0.039],[0.023,0.034],[0.013,0.02]\rangle $,
$\langle [0.065,0.092],[0.032,0.045],[0.021,0.032],[0.002,0.003]\rangle $,
$\langle [0.052,0.071],[0.017,0.024],[0.024,0.037],[0.029,0.052]\rangle $,
$\langle [0.032,0.044],[0.02,0.027],[0.011,0.015],[0.015,0.023]\rangle $,
$\langle [0.101,0.152],[0.06,0.062],[0.033,0.052],[0.018,0.021]\rangle $,
$\langle [0.085,0.12],[0.02,0.027],[0.023,0.033],[0.027,0.043]\rangle $,
$\langle [0.013,0.019],[0.006,0.008],[0.012,0.025],[0.005,0.009]\rangle $,
$\langle [0.027,0.041],[0.004,0.005],[0.004,0.006],[0.005,0.007]\rangle $,
$\langle [0.022,0.032],[0.01,0.013],[0.006,0.009],[0.006,0.009]\rangle $,
$\langle [0.074,0.105],[0.06,0.087],[0.017,0.024],[0.002,0.002]\rangle )$.
Then, Euclidean and Hamming distances to negative-ideal solution are calculated as in Table
7.
Table 7
Euclidean and Hamming distances to negative-ideal solution.
|
SO1 |
SO2 |
SO3 |
SO4 |
EN1 |
EN2 |
EN3 |
EC1 |
EC2 |
EC3 |
EC4 |
TE1 |
TE2 |
TE3 |
Euclidean distance |
AL1 |
0.0347 |
0.4797 |
0.4755 |
0.3479 |
0.0242 |
0.5786 |
0.5808 |
0.5999 |
0.0311 |
0.0367 |
0.3449 |
0.3510 |
0.0026 |
0.4939 |
|
AL2 |
0.0000 |
0.0652 |
0.3193 |
0.3504 |
0.3414 |
0.3524 |
0.0067 |
0.0178 |
0.0000 |
0.0133 |
0.0026 |
0.4881 |
0.3492 |
0.0000 |
|
AL3 |
0.0325 |
0.4800 |
0.3149 |
0.0000 |
0.5961 |
0.3445 |
0.0100 |
0.0041 |
0.0475 |
0.3389 |
0.3449 |
0.4881 |
0.4959 |
0.4733 |
|
AL4 |
0.0283 |
0.4808 |
0.5671 |
0.6978 |
0.4866 |
0.0045 |
0.0139 |
0.0241 |
0.4827 |
0.0368 |
0.3449 |
0.4881 |
0.4901 |
0.4535 |
|
AL5 |
0.6916 |
0.5865 |
0.0202 |
0.3475 |
0.3479 |
0.0066 |
0.0238 |
0.4830 |
0.0439 |
0.0368 |
0.3469 |
0.0004 |
0.0046 |
0.3237 |
|
AL6 |
0.0366 |
0.0611 |
0.0174 |
0.3503 |
0.0000 |
0.0066 |
0.5899 |
0.3457 |
0.0551 |
0.6689 |
0.4920 |
0.0065 |
0.3506 |
0.0338 |
|
AL7 |
0.0421 |
0.5903 |
0.0436 |
0.4927 |
0.0244 |
0.0312 |
0.4785 |
0.0013 |
0.5603 |
0.0327 |
0.4892 |
0.0106 |
0.0070 |
0.0156 |
|
AL8 |
0.0140 |
0.0000 |
0.3173 |
0.3477 |
0.4828 |
0.0000 |
0.0232 |
0.3448 |
0.3467 |
0.3408 |
0.4916 |
0.6086 |
0.0030 |
0.4937 |
Hamming distance |
AL1 |
0.1532 |
0.5260 |
0.5232 |
0.3796 |
0.1322 |
0.6087 |
0.6160 |
0.6218 |
0.1673 |
0.1773 |
0.3524 |
0.3537 |
0.0350 |
0.5115 |
|
AL2 |
0.0000 |
0.2474 |
0.4005 |
0.3764 |
0.3808 |
0.3538 |
0.0633 |
0.1245 |
0.0000 |
0.1013 |
0.0415 |
0.5010 |
0.3622 |
0.0000 |
|
AL3 |
0.1631 |
0.5306 |
0.3489 |
0.0000 |
0.6136 |
0.3880 |
0.0894 |
0.0510 |
0.1953 |
0.3600 |
0.3513 |
0.4999 |
0.5073 |
0.5044 |
|
AL4 |
0.1234 |
0.5376 |
0.6236 |
0.7113 |
0.5028 |
0.0458 |
0.0891 |
0.1474 |
0.5096 |
0.1446 |
0.3567 |
0.4995 |
0.5044 |
0.5014 |
|
AL5 |
0.7189 |
0.6299 |
0.1132 |
0.3641 |
0.3846 |
0.0668 |
0.1373 |
0.5117 |
0.1925 |
0.1446 |
0.3556 |
0.0135 |
0.0613 |
0.3631 |
|
AL6 |
0.1639 |
0.2388 |
0.1204 |
0.3729 |
0.0000 |
0.0668 |
0.6159 |
0.3639 |
0.2138 |
0.7029 |
0.4989 |
0.0623 |
0.3571 |
0.1597 |
|
AL7 |
0.1943 |
0.6372 |
0.1771 |
0.5090 |
0.1373 |
0.1451 |
0.5086 |
0.0247 |
0.6101 |
0.1505 |
0.5003 |
0.0901 |
0.0635 |
0.1031 |
|
AL8 |
0.0932 |
0.0000 |
0.3744 |
0.3719 |
0.5150 |
0.0000 |
0.1280 |
0.3733 |
0.3694 |
0.3856 |
0.4975 |
0.6155 |
0.0461 |
0.5017 |
At the final step, the relative assessment matrix is constructed, and scores are calculated (see Table
8).
Table 8
Relative assessment matrix and assessment scores based on HF-CODAS.
|
AL1 |
AL2 |
AL3 |
AL4 |
AL5 |
AL6 |
AL7 |
AL8 |
Scores |
Rank |
AL1 |
0 |
4.28 |
0.97 |
−0.36 |
2.22 |
2.59 |
2.87 |
1.45 |
14 |
2 |
AL2 |
−4.28 |
0 |
−3.31 |
−4.64 |
−2.06 |
−1.69 |
−1.41 |
−2.83 |
−20.2 |
8 |
AL3 |
−0.97 |
3.31 |
0 |
−1.32 |
1.25 |
1.62 |
1.9 |
0.49 |
6.29 |
3 |
AL4 |
0.36 |
4.64 |
1.32 |
0 |
2.58 |
2.94 |
3.23 |
1.81 |
16.9 |
1 |
AL5 |
−2.22 |
2.06 |
−1.25 |
−2.58 |
0 |
0.37 |
0.65 |
−0.77 |
−3.73 |
5 |
AL6 |
−2.59 |
1.69 |
−1.62 |
−2.94 |
−0.37 |
0 |
0.28 |
−1.13 |
−6.68 |
6 |
AL7 |
−2.87 |
1.41 |
−1.9 |
−3.23 |
−0.65 |
−0.28 |
0 |
−1.42 |
−8.93 |
7 |
AL8 |
−1.45 |
2.83 |
−0.49 |
−1.81 |
0.77 |
1.13 |
1.42 |
0 |
2.39 |
4 |
The results indicate that AL4 is the best alternative for construction site. The ranking of alternative sites is as follows: $AL4>AL1>AL3>AL8>AL5>AL6>AL7>AL2$.
5.3 Comparison with Ordinary Fuzzy CODAS and Hesitant TOPSIS Methods
In this sub-section, we compare our novel hesitant CODAS method with the ordinary fuzzy CODAS method. The membership values in the decision matrix of hesitant fuzzy CODAS are aggregated and thus, unified interval-valued fuzzy numbers are obtained to apply ordinary fuzzy CODAS method. Since trapezoidal fuzzy numbers are used in ordinary fuzzy CODAS method proposed by Keshavarz Ghorabaee
et al. (
2017), interval-valued fuzzy numbers are converted to trapezoidal fuzzy numbers. For instance, (3.15, 4.95) is converted to (3.15, 3.15, 4.95, 4.95). The aggregated decision matrix is given in Table
9.
Table 9
Decision matrix of ordinary fuzzy CODAS.
|
SO1 |
SO2 |
SO3 |
SO4 |
EN1 |
EN2 |
EN3 |
AL1 |
(3.15, 4.95) |
(4.05, 5.85) |
(4.73, 6.53) |
(4.5, 6.3) |
(3.6, 5.4) |
(1.8, 3.6) |
(1.58, 3.38) |
AL2 |
(1.35, 3.15) |
(4.05, 5.85) |
(4.5, 6.3) |
(4.28, 6.08) |
(2.7, 4.5) |
(3.85, 5.63) |
(4.05, 5.85) |
AL3 |
(3.6, 5.4) |
(4.28, 6.08) |
(3.15, 4.95) |
(1.35, 3.15) |
(2.48, 4.95) |
(2.25, 4.05) |
(3.6, 5.4) |
AL4 |
(2.48, 4.28) |
(4.5, 6.3) |
(5.18, 6.98) |
(5.18, 6.98) |
(3.15, 5.18) |
(4.5, 6.3) |
(4.05, 5.85) |
AL5 |
(6.08, 7.88) |
(4.73, 6.53) |
(2.93, 4.73) |
(3.15, 4.95) |
(2.7, 4.95) |
(4.05, 5.85) |
(3.15, 4.95) |
AL6 |
(3.6, 5.18) |
(4.05, 5.63) |
(3.15, 4.95) |
(4.05, 5.85) |
(5.2, 6.98) |
(4.05, 5.85) |
(1.8, 3.6) |
AL7 |
(4.5, 6.08) |
(4.95, 6.53) |
(3.6, 5.4) |
(4.05, 5.85) |
(3.38, 5.18) |
(3.15, 4.95) |
(2.25, 4.05) |
AL8 |
(2.25, 4.05) |
(1.58, 3.38) |
(3.83, 5.63) |
(3.83, 5.63) |
(2.25, 3.83) |
(4.73, 6.53) |
(3.6, 5.4) |
|
EC1 |
EC2 |
EC3 |
EC4 |
TE1 |
TE2 |
TE3 |
AL1 |
(1.8, 3.6) |
(4.5, 5.4) |
(2.93, 4.73) |
(3.6, 5.4) |
(4.73, 6.53) |
(4.95, 6.75) |
(2.73, 4.5) |
AL2 |
(3.38, 5.18) |
(6.18, 7.58) |
(3.83, 5.63) |
(3.83, 5.63) |
(2.48, 4.28) |
(2.93, 4.73) |
(5.63, 7.43) |
AL3 |
(4.95, 6.75) |
(3.83, 5.63) |
(4.05, 5.85) |
(4.05, 5.85) |
(2.93, 4.73) |
(2.48, 4.28) |
(2.48, 4.28) |
AL4 |
(3.15, 4.28) |
(3.38, 5.18) |
(3.83, 5.63) |
(2.93, 4.73) |
(2.7, 4.5) |
(2.48, 4.28) |
(2.48, 4.28) |
AL5 |
(2.03, 3.83) |
(3.83, 5.63) |
(3.83, 5.63) |
(2.93, 4.73) |
(5.4, 7.2) |
(4.28, 6.08) |
(3.15, 4.95) |
AL6 |
(3.38, 5.18) |
(3.6, 5.4) |
(1.58, 3.38) |
(2.93, 4.58) |
(4.5, 6.3) |
(3.6, 5.4) |
(3.15, 4.95) |
AL7 |
(5.18, 6.98) |
(2.7, 4.5) |
(3.38, 5.18) |
(2.48, 4.28) |
(2.93, 4.73) |
(4.28, 6.08) |
(4.05, 5.85) |
AL8 |
(2.7, 4.5) |
(4.05, 5.85) |
(2.93, 4.5) |
(3.38, 5.18) |
(2.03, 3.83) |
(4.5, 6.3) |
(3.18, 4.95) |
The results of the ordinary fuzzy CODAS method are given in Table
10.
Table 10
Relative assessment matrix and assessment scores for ordinary fuzzy CODAS method.
|
AL1 |
AL2 |
AL3 |
AL4 |
AL5 |
AL6 |
AL7 |
AL8 |
Scores |
Rank |
AL1 |
0.00 |
0.36 |
0.19 |
0.18 |
0.18 |
0.11 |
0.18 |
0.29 |
1.49 |
1 |
AL2 |
−0.36 |
0.00 |
−0.17 |
−0.18 |
−0.18 |
−0.25 |
−0.18 |
−0.08 |
−1.41 |
8 |
AL3 |
−0.19 |
0.17 |
0.00 |
0.00 |
−0.01 |
−0.08 |
0.00 |
0.10 |
−0.01 |
6 |
AL4 |
−0.18 |
0.18 |
0.00 |
0.00 |
0.00 |
−0.07 |
0.00 |
0.10 |
0.02 |
5 |
AL5 |
−0.18 |
0.18 |
0.01 |
0.00 |
0.00 |
−0.07 |
0.00 |
0.11 |
0.06 |
3 |
AL6 |
−0.11 |
0.25 |
0.08 |
0.07 |
0.07 |
0.00 |
0.07 |
0.17 |
0.59 |
2 |
AL7 |
−0.18 |
0.18 |
0.00 |
0.00 |
0.00 |
−0.07 |
0.00 |
0.11 |
0.05 |
4 |
AL8 |
−0.29 |
0.08 |
−0.10 |
−0.10 |
−0.11 |
−0.17 |
−0.11 |
0.00 |
−0.80 |
7 |
We apply different decision matrices since the different rankings are obtained from the ordinary fuzzy CODAS method. In most of the cases, both methods produced the same results. However, when the fuzziness is increased, our proposed method overcomes the disadvantages of the ordinary fuzzy sets and gives better solutions than the ordinary fuzzy CODAS method.
Secondly, we also compare our proposed method with hesitant fuzzy TOPSIS (Xu and Zhang,
2013). We used the same weighted normalized decision matrix since both methods have the same steps to obtain it. The positive ideal solutions of hesitant TOPSIS method are given in Table
11.
Table 11
Positive ideal solutions of hesitant TOPSIS method.
|
SO1 |
SO2 |
SO3 |
SO4 |
EN1 |
EN2 |
EN3 |
EC1 |
EC2 |
EC3 |
EC4 |
TE1 |
TE2 |
TE3 |
Sum |
AL1 |
0.671 |
0.448 |
0.433 |
0.585 |
0.674 |
0.349 |
0.323 |
0.316 |
0.63 |
0.635 |
0.603 |
0.602 |
0.695 |
0.494 |
7.46 |
AL2 |
0.692 |
0.618 |
0.533 |
0.588 |
0.577 |
0.579 |
0.672 |
0.672 |
0.658 |
0.658 |
0.695 |
0.491 |
0.595 |
0.704 |
8.73 |
AL3 |
0.668 |
0.442 |
0.575 |
0.698 |
0.473 |
0.553 |
0.667 |
0.686 |
0.618 |
0.57 |
0.604 |
0.492 |
0.479 |
0.497 |
8.02 |
AL4 |
0.678 |
0.431 |
0.304 |
0 |
0.479 |
0.675 |
0.667 |
0.669 |
0.434 |
0.649 |
0.599 |
0.493 |
0.486 |
0.498 |
7.06 |
AL5 |
0 |
0.313 |
0.648 |
0.598 |
0.569 |
0.673 |
0.655 |
0.473 |
0.619 |
0.649 |
0.601 |
0.697 |
0.693 |
0.609 |
7.8 |
AL6 |
0.669 |
0.625 |
0.646 |
0.591 |
0.687 |
0.673 |
0.314 |
0.586 |
0.609 |
0 |
0.491 |
0.693 |
0.599 |
0.701 |
7.88 |
AL7 |
0.659 |
0.302 |
0.63 |
0.485 |
0.669 |
0.656 |
0.457 |
0.688 |
0.323 |
0.644 |
0.49 |
0.688 |
0.692 |
0.703 |
8.09 |
AL8 |
0.684 |
0.677 |
0.556 |
0.592 |
0.47 |
0.677 |
0.657 |
0.579 |
0.547 |
0.55 |
0.492 |
0.333 |
0.694 |
0.496 |
8 |
The negative ideal solutions of the hesitant TOPSIS method are given in Table
12.
Table 12
Positive ideal solution of hesitant TOPSIS method.
|
SO1 |
SO2 |
SO3 |
SO4 |
EN1 |
EN2 |
EN3 |
EC1 |
EC2 |
EC3 |
EC4 |
TE1 |
TE2 |
TE3 |
Sum |
AL1 |
0.035 |
0.48 |
0.476 |
0.348 |
0.021 |
0.579 |
0.581 |
0.6 |
0.032 |
0.037 |
0.345 |
0.351 |
0.003 |
0.499 |
4.39 |
AL2 |
0 |
0.065 |
0.319 |
0.35 |
0.341 |
0.352 |
0.007 |
0.018 |
0 |
0.013 |
0.003 |
0.488 |
0.349 |
0 |
2.31 |
AL3 |
0.032 |
0.48 |
0.315 |
0 |
0.486 |
0.345 |
0.01 |
0.004 |
0.048 |
0.339 |
0.345 |
0.488 |
0.496 |
0.498 |
3.88 |
AL4 |
0.028 |
0.481 |
0.567 |
0.698 |
0.487 |
0.004 |
0.014 |
0.024 |
0.483 |
0.037 |
0.345 |
0.488 |
0.49 |
0.496 |
4.64 |
AL5 |
0.692 |
0.586 |
0.02 |
0.348 |
0.347 |
0.007 |
0.024 |
0.483 |
0.044 |
0.037 |
0.347 |
0.0004 |
0.005 |
0.351 |
3.29 |
AL6 |
0.037 |
0.061 |
0.017 |
0.35 |
0 |
0.007 |
0.59 |
0.346 |
0.055 |
0.669 |
0.492 |
0.006 |
0.351 |
0.003 |
2.98 |
AL7 |
0.042 |
0.59 |
0.044 |
0.493 |
0.023 |
0.031 |
0.479 |
0.001 |
0.56 |
0.033 |
0.489 |
0.011 |
0.007 |
0.001 |
2.8 |
AL8 |
0.014 |
0 |
0.317 |
0.348 |
0.483 |
0 |
0.023 |
0.345 |
0.347 |
0.341 |
0.492 |
0.609 |
0.003 |
0.499 |
3.82 |
After the calculations, results of the hesitant TOPSIS method are calculated as in Table
13.
Table 13
Positive ideal solution of hesitant TOPSIS method.
|
AL1 |
AL2 |
AL3 |
AL4 |
AL5 |
AL6 |
AL7 |
AL8 |
Score |
0.37 |
0.21 |
0.33 |
0.4 |
0.3 |
0.27 |
0.26 |
0.32 |
Rank |
2 |
8 |
3 |
1 |
5 |
6 |
7 |
4 |
The results of the compared methods are the same. Thus, our proposed model is valid where hesitant fuzzy sets can be used as input data.
5.4 Sensitivity Analysis
One-at-a-time sensitivity analysis based on each criterion is performed to demonstrate the effects of changes on the results. To visualize this analysis, we develop a pattern which is given in Table
14. After the changes on weights of the sub-criteria through this pattern, hesitant fuzzy CODAS method operations are re-processed. In Table
14, only the first and second alternatives are presented.
Table 14
Pattern for the sensitivity analysis.
Pattern |
Sets with respect to criteria |
Test variables |
SO1 |
SO2 |
TE3 |
0.1 |
AL4, AL1 |
AL4, AL1 |
AL4, AL1 |
0.2 |
AL4, AL1 |
AL4, AL1 |
AL4, AL1 |
0.3 |
AL4, AL1 |
AL4, AL1 |
AL4, AL1 |
0.4 |
AL4, AL1 |
AL4, AL1 |
AL4, AL1 |
0.5 |
AL4, AL1 |
AL4, AL1 |
AL4, AL1 |
0.6 |
AL4, AL1 |
AL4, AL1 |
AL4, AL1 |
0.7 |
AL4, AL1 |
AL4, AL1 |
AL4, AL1 |
0.8 |
AL4, AL1 |
AL4, AL1 |
AL4, AL1 |
0.9 |
AL4, AL1 |
AL4, AL1 |
AL4, AL1 |
1.0 |
AL4, AL1 |
AL4, AL1 |
AL4, AL1 |
When the results of the sensitivity analyses are examined, it is revealed that criterion SO2 with an interval of $[0.7,1]$ and criterion EC3 with an interval of $[0.7,1]$ affect the results. But they don’t affect the rank of the best alternative. This verifies the robustness of the proposed model on the given decision.