1 Introduction
Green communication demonstrates the environmentally friendly practices of businesses and the strategies they employ to promote their products to customers. In this process, it is aimed to achieve sustainability targets. Green communication is important for businesses in many ways. First, it is possible to achieve customer satisfaction owing to environmentally friendly practices (Dev
et al.,
2022). This helps meet the expectations of consumers who are especially sensitive to environmental issues. Thus, businesses can increase customer loyalty more easily. On the other hand, thanks to successful green communication, businesses can gain a significant competitive advantage. Businesses that pay more attention to environmental factors may be more preferred by customers (Tewari
et al.,
2022). In addition, thanks to green communication, it enables businesses to act more in accordance with legal procedures. This can minimize the possibility of paying financial penalties due to illegal practices.
There are some effective issues that businesses should take into consideration to increase their green communication performance. First, green communication must be carried out in a transparent and honest manner. In this context, it is important for businesses to share realistic information with their customers in this process. On the other hand, in this regard, the issues communicated by businesses regarding sustainability must be documented (Kumar
et al.,
2022). This allows the credibility of the activities carried out to be increased. In addition, green communication activities must meet customer expectations. In this context, the basic needs of customers should be clearly determined. Communication activities prepared for these needs also help businesses to be successful. Thus, customer satisfaction can increase, which provides businesses with a significant competitive advantage (Haripriya
et al.,
2022). Furthermore, financial issues should also be taken into consideration for the effectiveness of the green communication practices.
It is very necessary to carry out green communication activities effectively to increase the performance of the business. Despite this situation, businesses have very limited resources such as budget and manpower. Therefore, taking many actions to increase green communication performance is not financially feasible as it will increase costs. It is necessary to conduct a priority analysis on the issues that affect the performance of green communication activities of businesses (Sousa
et al.,
2022). On the other hand, this priority analysis helps the business define its green communication goals more clearly. In this way, it is possible to implement more accurate strategies in a timely manner. However, there are very few scientific studies on this subject in the literature. Instead, studies generally appear to emphasize important factors affecting the effectiveness of this process. This situation is accepted as the main gap in the literature. In other words, there is a significant need for a priority analysis on the variables that will affect the green communication performance of businesses.
Accordingly, this study aims to evaluate the main determinants of the green communication effectiveness of the companies. Within this context, a novel fuzzy decision-making model has been constructed in this study that has two main different stages. Firstly, selected indicators are examined by using artificial intelligence-based sine trigonometric Pythagorean fuzzy DEMATEL. On the other side, at the second stage, emerging seven countries are ranked according to the performance of the green communication. For this purpose, artificial intelligence-based sine trigonometric Pythagorean fuzzy RATGOS technique is taken into consideration. In addition to this condition, these countries are also ranked by using ARAS methodology to make a comparative evaluation. The main motivation of making this study is the need for a novel decision-making model. The main reason is that existing similar models in the literature could not consider the weights of the decision makers. These people can have difficult qualifications according to their demographic factors. Due to this issue, the opinions of the more qualified decision maker should be considered with a higher weight of importance. To achieve this goal, the decision matrix in this proposed model is obtained using an artificial intelligence approach. This allows decision makers to determine their importance weights according to their qualifications. In this way, it is possible to make more accurate evaluations.
The main contributions of this study are demonstrated below.
-
(i) Green communication activities play a critical role for the performance improvement of the companies. However, because it is quite difficult to define appropriate strategies for this situation, a comprehensive decision-making model should be generated to reach this objective. Most of the studies in the literature focused on the main performance indicators of the green communication effectiveness. However, because of the limited budget of the companies, many improvements cannot be performed. In other words, for the purpose of cost effectiveness, necessary actions should be taken for prior factors. In this context, there is a strong need for new models that can handle uncertainty problem more effectively. Because of this need, a novel and detailed fuzzy decision-making model has been created in this study.
-
(ii) Artificial intelligence methodology is integrated with the fuzzy decision-making model. In most of the previously generated decision-making model, the opinions of all experts are taken into account with the same weight of importance (Ecer
et al.,
2024; Alamoodi
et al.,
2024; Mikhaylov
et al.,
2024). However, this situation is criticized by many different scholars (Kou
et al.,
2024; Ebrahimi
et al.,
2024). To overcome this problem, artificial intelligence methodology is considered to generate decision matrix. In other words, the evaluations can be evaluated based on the working experience of the experts. With the help of this situation, more appropriate calculations can be made.
-
(iii) Proposing RATGOS methodology to the literature by the authors is another significant contribution of this proposed model. There are some important criticisms to the existing ranking decision-making techniques in the literature (Bánhidi and Dobos,
2024). For instance, in the analysis of TOPSIS method, Euclidean distance is taken into consideration with respect to the calculation of the negative ideal solution (Trivedi
et al.,
2024; Tüysüz and Kahraman,
2024). For the purpose of handling these drawbacks, RATGOS model is generated by making computations with geometrical mean. In this context, it can be possible to reach more effective solutions.
-
(iv) Making calculations by using sine trigonometric fuzzy sets also provides some advantages. The main superiority of these sets is that the values can be defuzzified in a more effective manner. This situation has a positive contribution to the minimization of the uncertainty. In addition to this issue, sine trigonometric fuzzy sets also consider periodicity in the analysis process (Cali
et al.,
2024). Owing to this condition, more appropriate findings can be achieved. Furthermore, expressiveness in handling relationships can be increased with the help of sine trigonometric fuzzy numbers (Palanikumar
et al.,
2024). This situation can be accepted as the main superiority over intuitionistic vague fuzzy sets (IVIFS) or q-rung orthopair fuzzy sets (qROF) (Shahzadi
et al.,
2024). Similarly, making a comparative evaluation by ARAS also brings some benefits. This situation helps to measure the reliability of the findings of the proposed model.
-
(v) Considering DEMATEL methodology provides some advantages for the performance of the proposed model. By using DEMATEL technique, complex relationships among the indicators can be handled more effectively (Pandey
et al.,
2024). However, other methods like LBWA (Linear Barycentric Weighting Approach) and BWM (Best-Worst Method) do not focus on this situation (Shanta
et al.,
2024). In addition to them, causal relationship between these factors can be taken into consideration while making evaluation via DEMATEL methodology. However, other methods, such as FUCOM (Fuzzy Compromise Solution) or DIBR (Direct and Indirect Benefits and Costs) cannot make this kind of evaluation (Ghorbani
et al.,
2024).
The second section provides information about the literature review. Thirdly, methodology is explained. In the fourth part, the results are highlighted. The final parts include conclusion and discussion.
2 Literature Review
In the literature study on the concept of green advertising, it is seen that the concept of trust is at the forefront. It is stated that the element of trust has an impact on many issues, from consumers’ skepticism to advertising, to their persuasion, and from purchasing behaviour to brand loyalty (You
et al.,
2022). Thus, ensuring trust in green advertising provides many benefits to advertisers (Li
et al.,
2022). Since trust brings credibility, consumers who believe in green advertising will be more convinced to buy the product. A green advertisement that provides this condition will be approached with complete trust rather than skepticism (Hsu
et al.,
2022). Approaching them with confidence also makes it easier for them to accept the message coming from that advertisement. However, green advertising skepticism can hinder the intention to purchase green products (Dogra
et al.,
2022). Trust, on the other hand, completely mediates the relationship between skepticism and purchase intention (de Sio
et al.,
2022). Verleye
et al. (
2023) examined to what extent green advertising is trusted by consumers in Belgium. They state that green advertising will increase trust with six elements: honesty, openness, detail, commitment, urgency, and shared values. Khandelwal and Singh (
2023) defined the effect of repetition of green advertising in the media on the trust factor. The frequency of repetition of the advertisement increases the trust in green advertising and has a positive effect on the trust in the brand, advertising message and advertisers. Additionally, Zhang and Yang (
2023a) evaluated the element of trust in green advertising through the concept of brand loyalty. They also emphasized that attractiveness and informativeness elements in green advertising have a positive impact on brand loyalty.
The environmental issue is one of the most important criteria in increasing the success of green advertising. The environmental problem experienced on a global scale has increased the importance of the concept of sustainable development in business strategy and therefore obliges businesses to offer green products to the market (Dai and Sheng,
2022). Advertisements prepared with effective green advertising strategies motivate consumers to consume green products. Attraction with high awareness of environmental responsibility and benefits can encourage consumers to purchase green products (de Sio
et al.,
2022). Nguyen-Viet (
2022) stated that individuals are increasingly aware of the environmental consequences of consumption. In this process, it is pointed out that eco-labels affect the purchasing behaviour and positively affect the brand value. Tu
et al. (
2022) investigated the role of the internet of things and green advertisements in consumer behaviour. They concluded that green advertising increases consumers’ awareness of environmental protection and has an impact on their behaviour. Zeng
et al. (
2022) analysed the effect of green advertising on low carbon awareness, emphasizing that consumers do not value low-carbon products as they should. It is concluded that green advertising plays a role in the adoption of remanufactured products and a low-carbon supply chain. Hartmann
et al. (
2023) defined that the advertising industry encourages consumers to consume and that causes climate change. The importance of green advertising is extremely important in helping consumers move from climate-damaging consumption behaviour to low-carbon behaviour.
Informativeness is a criterion that is valued for businesses to implement a successful green advertising application. A green advertising message with a strong informative aspect has a persuasive effect that directs the consumer to purchase (Riyal
et al.,
2022; Gupta
et al.,
2022). In this orientation, the attractiveness, as well as the usefulness of the information contained in green advertising, is extremely effective in purchasing and sharing behaviour (Wang and Li,
2022). Zhang and Yang (
2023b) denoted that the informativeness element affects the trust factor in green advertising and leads to green brand love. Hartmann
et al. (
2023) pointed out that green advertising can create a positive awareness in the consumer’s mind with correct information and moral satisfaction. Correct information increases the effectiveness of green advertising among consumers. Ni
et al. (
2022) examined the concept of comparative advertising, which is neglected in research in the promotion of green products. Comparative advertisements lead to more positive purchasing behaviour than other advertisements and have a positive effect on consumers with low green involvement (Wang
et al.,
2022).
Another criterion that is extremely important for green advertising in increasing advertising investments is financial issues. Companies need to invest in green advertising to reduce the environmental impacts caused during production stages and to achieve their sustainability targets (Xu
et al.,
2022). It is seen that advertising investments increase the profit of the manufacturer who produces brown and green products at the same time and greatly increase the greening levels of the products (Shi
et al.,
2022). Zhang
et al. (
2022) examined the green advertising investments of fashion manufacturers and rental platforms. While fashion companies that invest in green advertising earn high profits, companies that do not invest continue their lives with low profitability (Fahad and Hburi,
2022). Consumer sensitivity to green advertising explains this profit balance. This situation shows the necessity of investing in green advertising for fashion manufacturers to have effective success in the rental market. Li
et al. (
2023) brought a different perspective by investigating the impact of religious dimensions on green advertising’s purchasing behaviour. They investigated Muslims’ reactions to green advertisements and their religious and cultural influences on purchasing. As a result of the study, it is seen that religious attractiveness elements positively mediate green product purchasing behaviour. Ktisti
et al. (
2022) discussed the concepts of green advertising and green marketing through social media. It is emphasized that consumers use social media effectively in the process of searching and purchasing a product and that the effect of social media is neglected in green advertising research (Amoako
et al.,
2022).
By considering these issues, it is understood that there are some critical indicators of the green communication performance. Reliability is of great importance for green communication to be successful. In this way, the image of the transactions in the eyes of consumers can be improved. This allows products to be sold more easily. Transparency means that businesses share their environmentally friendly practices honestly and understandably. Customers can make more informed choices thanks to the environmental information they receive transparently. Informativeness is a very important element for the success of green communication. As consumers are informed about their environmental responsibilities, they can make more conscious choices. Attractiveness helps green communication be effectively communicated and received. This allows the message to be understood better. Meeting customer expectations supports the effective implementation of green communication. Therefore, businesses can gain competitive advantage by meeting these expectations. On the other hand, quality products and services contribute to increasing customer satisfaction. Thus, green communication activities can be more successful. Financial success enables businesses to improve their sustainability projects. In this way, it is possible to implement more successful green communication activities. In addition to this issue, graphical representation results of the keywords are also obtained. In this framework, the articles published in 2023 with the subject of “green communication” are provided from the Web of Science platform. A keyword analysis has been performed to understand the main relationship between the factors. The details of the results are illustrated in the appendix part (Fig.
A1). This figure gives information that the critical term in this framework is the innovation. Thus, by creating innovative strategies, companies can integrate digitalization with the sustainable issues. In other words, it is seen that innovation is quite necessary for the companies to improve the performance of green communication.
As a result of the literature review, it is possible to reach the following conclusions. Green advertising is extremely important in terms of increasing profitability for businesses by improving the sales of green products. The fact that the primary responsibility of businesses is economic responsibility causes consumers to approach green advertising with skepticism. The main reason why consumers do not trust is the idea that businesses make green advertisements to make profit and therefore contain misleading information. Therefore, accurate, transparent and clear information must be provided to increase reliability among consumers. Consumers who are informed with accurate and transparent information will tend to be interested in green products. Effective green advertising practices can prevent global crises and climate changes by encouraging consumers to consume green. Therefore, as the trust, informativeness, solution to environmental problems and financial investments in green advertising increase, its effect on consumers and businesses becomes more successful. Trust positively affects consumers’ purchasing decisions, and it is known that companies that fulfill their environmental responsibilities are more trusted. Therefore, environmental responsibility and accurate information brings trust with it. This situation offers more investment opportunities to businesses in the long term. Being able to invest more in green advertising means making these ads more effective, creative and attractive. When all these elements are met, green advertising will have a positive impact on purchasing behaviour and will provide a long-term profit advantage to businesses. Improving these factors is vital for increasing the green communication performance of businesses.
On the other hand, improvements made for these factors also cause the costs of businesses to increase. Therefore, a priority analysis needs to be made for these factors so that businesses can make improvements towards more important variables. For this purpose, a novel multi-criteria decision-making model should be generated to find the most critical factors of green communication effectiveness. These indicators can have an influence on each other. Because of this issue, the causal relationship between the items should be taken into consideration to make more effective evaluation. Another critical issue in this framework is that the qualification of the decision-makers can be different. Due to this issue, in a new model, the weights of these people should be computed according to their demographical factors. Moreover, existing ranking techniques like TOPSIS and VIKOR are criticized because of some situations. Thus, there is a strong need for a new ranking technique. By considering these needs, in this study, a new decision-making model is developed to identify the main performance indicators of green communication. Firstly, selected indicators are examined by using artificial intelligence-based sine trigonometric Pythagorean fuzzy DEMATEL. In this process, artificial intelligence methodology is considered to compute the weights of the decision makers. Similarly, with the help of considering DEMATEL, the causal relationship between the criteria can be determined. On the other side, at the second stage, emerging seven countries are ranked according to the performance of the green communication. For this purpose, artificial intelligence-based sine trigonometric Pythagorean fuzzy RATGOS technique is taken into consideration. In this context, to overcome the criticisms to existing ranking techniques, RATGOS methodology is proposed by making computations with geometrical mean. In this context, it can be possible to reach more effective solutions.
3 Proposed Model
The proposed model consists of two different stages. The first stage is about weighing the factors affecting green communication. For this purpose, artificial intelligence-based sine trigonometric Pythagorean fuzzy DEMATEL method is used. In the second stage, emerging seven countries are ranked based on the criteria. In this direction, artificial intelligence-based sine trigonometric Pythagorean fuzzy RATGOS method is taken into consideration. The steps of this two-stage proposed method are shown in Fig.
1.
The details of the methods used in this proposed model are explained in the following subtitles.
3.1 Obtaining Decision Matrices with Artificial Intelligence
Decision matrixes used in multi-criteria decision-making methods are obtained using artificial intelligence in this proposed model. In Step 1, a mathematical model for criteria weighting within the framework of artificial intelligence is constructed. This model’s creation is executed utilizing the Keras and TensorFlow libraries in the Python programming language. The selection of this software is grounded in its open-source nature and its remarkable data analysis capabilities. Expert opinions and experience constitute the input layer of the model. In other words, the designed artificial intelligence model has two inputs. These are the expert’s evaluation and the expert’s experience. Another component of artificial intelligence is the hidden layer structure. This layer provides the model with a non-linear structure. Thus, the linear relationship between the experience of the experts and the importance level of the expert is eliminated. It is important to note that an expert with 30 years of experience does not possess evaluations that are merely twice as significant as those of an expert with 15 years of experience. Therefore, a nonlinear structure is preferred to obtain the decision matrix (Zhang
et al.,
2023). The number of hidden layers supports the nonlinear structure. When artificial intelligence studies are examined, it is seen that models are built with different numbers of hidden layers, but the mostly preferred number of layers has been determined as “7”. To enhance the depth of these designated layers, an allocation of 128, 64, 32, 16, 8, 4, and 2 neurons is systematically introduced. This strategic augmentation is intended to amplify the learning capabilities of the artificial intelligence model. Since fuzzy numbers are generated from expert opinions and experiences, the Sigmoid function is preferred as the activation function of the hidden layers. The Sigmoid function, as defined in equation (1), incorporates the mathematical constant, “
e”, (approximately 2.71828182) and the parameter “
a” that characterizes the slope of the Sigmoid function. Within this equation, “
x” represents the variable subject to transformation.
The activation function is the function that changes the sum of the inputs in the neurons in the layers. In the literature, various activation functions have been described. It is preferred why the target set of the sigmoid function is between 0 and 1. Thus, it is aimed to ensure the condition of the membership value of fuzzy numbers. Finally, the output layer of artificial intelligence is designed. Since sine trigonometry has two components of Pythagorean fuzzy numbers, a two-output artificial intelligence model is planned.
Step 2 delves into the realm of machine learning within the context of artificial intelligence. This step is the calculation of the parameters of the model designed in the previous step. Adam algorithm is used to calculate the values of the connection between neurons. The optimization algorithm was put forward in 2015. This algorithm is frequently preferred in the field of deep learning because it gives good results quickly. It is also a computationally efficient algorithm due to its small memory requirement. The mathematical details of the algorithm are given in equations (
2)–(
6) (Reyad
et al.,
2023).
In this context,
${W_{t}}$ represents the parameter of
tth iteration,
a demonstrates learning coefficient,
w gives information about the weight,
β indicates the degree to which past gradients are involved in the process and
$\frac{\partial L}{\partial {w_{t}}}$ refers to the gradient. Moreover,
S and
V give information about the randomly generated initial values. A training data set is used for artificial intelligence to learn. The input data in this data set is randomly generated by simulation. The output data of the data set is obtained using equations (
7) and (
8).
In this context,
d in the equations refers to the experience periods of the experts. Moreover,
${\mu _{i}}$ indicates the membership value and
v refers to the non-membership value. Furthermore,
n demonstrates the number of experts. The values in Table
1 are considered for the fuzzification of the numbers (Giri
et al.,
2022).
Table 1
Linguistic variables for weighting.
Score |
Linguistic variables |
Fuzzy numbers |
$\sin (\frac{\pi }{2}\mu )$ |
$\sqrt{1-{\sin ^{2}}\big(\frac{\pi }{2}\sqrt{1-{v^{2}}}\big)}$ |
1 |
Absolutely low (AL) |
.2334 |
.5136 |
2 |
Low (L) |
.3827 |
.3716 |
3 |
Fairly low (FL) |
.5225 |
.2651 |
4 |
Medium low (ML) |
.7071 |
.1187 |
5 |
Fairly high (FH) |
.8526 |
.0702 |
6 |
High (H) |
.9239 |
.0353 |
7 |
Absolutely high (AH) |
.9724 |
.0126 |
The fuzzy weighted average of the expert opinions, serving as the output variable of the artificial intelligence model, is a crucial component of the decision-making process. The train process between input and output variables is repeated 100 times. In other words, the epoch number of the model is defined as 100. Since it is seen that more repetition does not improve the learning rate, this value is preferred. In other words, it is observed that increasing the number of epochs does not reduce the error value of the model.
Regarding Step 3, the validation test of the developed artificial intelligence is carried out. In this context, the outputs obtained with the test data are compared with the actual values. The mean squared error (MSE) is calculated for this comparison by using equation (
9).
Within this framework, Y represents the actual value and $\hat{Y}$ is the output value of the model. The MSE value calculated in this study is “.0023”. On the other hand, m shows the amount of data. Owing to this created and tested artificial intelligence, a fuzzy decision matrix is obtained by considering expert opinions and experience periods. This situation allows for more accurate analysis with the non-linear contribution of the weight of experts’ experience period.
3.2 Sine Trigonometric Pythagorean Fuzzy DEMATEL
DEMATEL is a valuable tool for weighting criteria in multi-criteria decision-making processes. It makes calculation by considering the interactions and impacts between criteria to determine their respective weights (Paul
et al.,
2023). The steps of this method with sine trigonometric Pythagorean fuzzy numbers are given below.
Step 1: Evaluations are obtained from the expert team.
Step 2: The fuzzy decision matrix $(D)$ is obtained using artificial intelligence.
Step 3: The values are defuzzied with equation (
10). Defuzzied decision matrix
$(A)$ is shown by equation (
11).
Step 4: With equations (
12) and (
13), a normalized direct-relation matrix
$(X)$ is computed.
Step 5: Total relation matrix (
T) is calculated with the help of equation (
14).
Step 6: The row (
R) and column (
D) sums of this matrix are constructed by equations (
15) and (
16).
Step 7: Using row and column totals, weights (
w) are calculated by equation (
17).
3.3 Sine Trigonometric Pythagorean Fuzzy RATGOS
RATGOS is a ranking method for alternatives according to criteria. This new technique is proposed by the authors to overcome the criticisms related to the existing ranking techniques (Oflaz
et al.,
2023). The basis of this method is the geometric mean of the similarity ratio to the optimal value (Dinçer
et al.,
2023a,
2023b). The steps of the RATGOS method with sine trigonometric Pythagorean fuzzy numbers are following.
Step 1: The evaluations of the experts are taken.
Step 2: Sine trigonometric decision matrix (STM) is created by using artificial intelligence approach.
Step 3: The defuzzification matrix (
A) is calculated by equation (
10). Next, optimal values are computed with equation (
19).
Step 4: With the help of equations (
20) and (
21), each criterion is divided by the optimal value. Thus, the similarity ratio matrix
$(N)$ is computed.
Step 5: The weighted similarity ratio matrix (
Z) is calculated by multiplying with the weights with equation (
22). In this scope,
w values show the weights of the criteria.
Step 6: The average similarity ratio is calculated by taking the geometric mean (
G) of values in
N matrix for each alternative by equation (
23).
3.4 Sine Trigonometric Pythagorean Fuzzy ARAS
The ARAS method is another ranking method used in multi-criteria decision analysis, similar to RATGOS. The main feature of this method is that it calculates the total ratio. The first two steps of RATGOS are similar in the ARAS.
Step 1: The evaluations of the experts are taken.
Step 2: Sine trigonometric decision matrix (STM) is created by using artificial intelligence approach.
Step 3: The optimal values of the decision matrix determined by artificial intelligence are calculated. For this purpose, score values are calculated with equation (
10). For benefit criteria, the criterion value with the higher score is determined as the optimal value (Nithyanandham and Augustin,
2023). For cost criteria, the value of the criterion with the lowest score is determined as the optimal value.
Step 4: In the next step, the weighted decision matrix
$(K)$ is obtained by multiplying the decision matrix and the weights. All values of the decision matrix, including the optimal values, are multiplied by the weights. Equation (
25) is used for this multiplication operation (Mishra and Rani,
2023).
Step 5: The weighted decision matrix and the optimal value are defuzzied by equation (
10). Then, row totals
$(S)$ are taken for each alternative and optimal with the help of equation (
26).
Step 6: The
Q values are calculated by dividing the total values by the optimal sum as in equation (
27). The larger
Q value is considered the most optimal value.
In this equation,
${S_{0}}$ is the sum of optimal values and
${S_{i}}$ denotes the sum of values for the
ith alternative.
4 Results
This study is aimed to evaluate the main indicators to increase green communication performance of the companies. For this purpose, a novel artificial intelligence-based fuzzy decision-making model is proposed. This model consists of two different stages. In the first stage, selected indicators are examined to find the significance weights. Secondly, the group of seven countries are ranked regarding the success of green communication. In the analysis process, the evaluations of three experts are taken into consideration. These people are academicians and top managers that have sufficient working experience in this area. The opinions of these people are taken via online meetings. The results of the proposed methods are summarized in the following subtitles.
4.1 Weighting the Criteria with Artificial Intelligence-Based Sine Trigonometric Pythagorean Fuzzy DEMATEL
In the first stage, selected variables are weighted. For this situation, Step 1 involves the establishment of the mathematical framework underpinning the artificial intelligence model used for weight determination. Step 2 entails the execution of machine learning techniques to ascertain the parameters governing the artificial intelligence model. In the final phase, Step 3, the trained artificial intelligence’s validity is assessed. The computed average error for the weight-designed artificial intelligence stood at a remarkably low value of .0023. This result confirms the validity due to its proximity to zero. Consequently, based on the integration of expert opinions and experience throughout this research, there exists no impediment to constructing a decision matrix derived from the artificial intelligence model.
Step 4 is related to expert opinions and experience periods. In this process, seven different criteria are defined based on the literature review results. These factors are detailed in Table
2.
Criteria |
Codes |
Reliability |
Reliability |
Transparency |
Transparency |
Informativeness |
Informativeness |
Attractiveness |
Attractiveness |
Meeting the Expectations of Target Customer |
TargetCustomer |
Quality of the Goods and Services |
Quality |
Financial Issues |
Financial |
In this framework, opinions are collected from three different experts with their experience periods. These experts’ experience periods are 17, 27 and 23 years. Additionally, experts’ evaluations are given in Table
A1.
In
Step 5, decision matrix is created using artificial intelligence with experts’ opinions in Table
A1 and their experience periods. The decision matrix obtained using artificial intelligence is shown in Table
A2.
Step 6 is about normalizing the decision matrix. For this issue, the fuzzy decision matrix is defuzzied with equation (
10). Then,
A matrix is normalized by equations (
12) and (
13). The normalized direct-relation matrix (
X) is given in Table
A3.
In
Step 7, the total relationship matrix is generated. Using the normalized direct relationship matrix, the total relationship matrix is calculated with the help of equation (
14). This matrix is shown in Table
A4. In this process, expert opinions and the years of working experience are defined as input variables to the artificial intelligence system. After this, sine trigonometric Pythagorean fuzzy decision matrix is obtained by running the model.
In the
Step 8, the weights of the criteria are obtained. First, the row and column totals of the total relationship matrix are calculated with the help of equations (
15) and (
16). Using these totals, the weights of the criteria are determined. For this situation, equation (
17) is considered. The weight values are given in Table
3.
Table 3
Weights of criteria.
Criteria |
Weights |
Reliability |
.1420 |
Transparency |
.1415 |
Informativeness |
.1456 |
Attractiveness |
.1425 |
TargetCustomer |
.1447 |
Quality |
.1425 |
Financial |
.1412 |
The ranking results of these criteria are shown in Fig.
2.
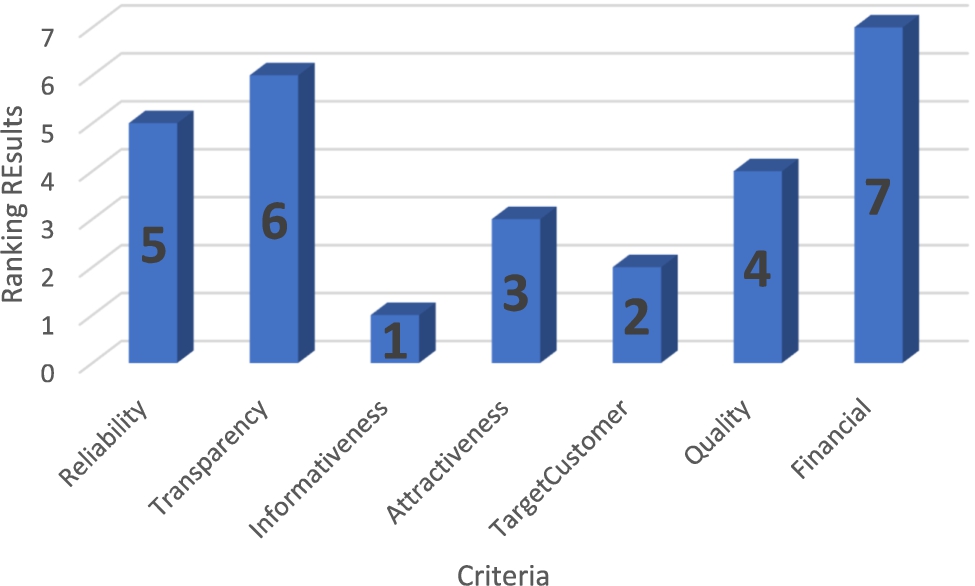
Fig. 2
Ranking results of the criteria.
Both Table
3 and Fig.
2 demonstrate that the most significant criterion is “Informativeness”. This determination is primarily substantiated by its notable weight value, which stands as the highest among all criteria at .1456. On the other side, the second most critical factor in this respect is meeting the customer expectations. However, transparency and financial issues have lower significance.
4.2 Ranking the Alternatives with Artificial Intelligence-Based Sine Trigonometric Pythagorean Fuzzy RATGOS
Stage 2 is about ranking the alternatives that are defined as emerging seven countries (Brazil, China, India, Indonesia, Mexico, Russia, Turkey). Step 9 is the mathematical construction of the artificial intelligence model required for ranking. This step bears a resemblance to the procedure carried out in Step 1. A structure comprising seven hidden layers and employing a Sigmoid activation function has been formulated. Thus, a non-linear model is designed that takes into account expert opinions and experience periods.
In
Step 10, data training is performed to artificial intelligence. For this purpose, the necessary algorithm for calculating the parameters is created by using equations (
2)–(
6). The data set for training the created artificial intelligence is obtained by simulation. Based on this data set, the output variable is calculated with equations (
7) and (
8). However, the sine trigonometric Pythagorean fuzzy numbers in Table
4 are taken into consideration in this process.
Table 4
Linguistic variables for ranking.
Score |
$\sin (\frac{\pi }{2}\mu )$ |
$\sqrt{1-{\sin ^{2}}(\frac{\pi }{2}\sqrt{1-{v^{2}}})}$ |
1 |
.2334 |
.7273 |
2 |
.3827 |
.6063 |
3 |
.4540 |
.5136 |
4 |
.5225 |
.3716 |
5 |
.6494 |
.2651 |
6 |
.8090 |
.0927 |
7 |
.8910 |
.0702 |
8 |
.9511 |
.0511 |
9 |
.9969 |
.0224 |
The model is trained on the input and output variables obtained by simulation. During the training process, 100 epochs have been applied. In
Step 11, the artificial intelligence model developed for ranking is tested. For this purpose, the MSE value in equation (
9) is defined that is calculated as .017. In other words, the squared mean of the difference between the actual values and the model’s output values is very close to 0. Therefore, it can be stated that the model is valid.
Step 12 is about obtaining the decision matrix with expert opinions and experience periods. Opinions are obtained from three different experts with 17, 27 and 23 years of experience, respectively. Expert opinions are indicated in Table
A5.
Step 13 is related to the creation of the decision matrix with the use of artificial intelligence. Experts’ opinions and experience periods are given as input to artificial intelligence. Using this data, the decision matrix is obtained from artificial intelligence. Decision matrix for ranking is denoted in Table
A6.
Step 14 includes the creation of the similarity ratio matrix and the weighted similarity ratio matrix. For this issue, by using equation (
10), the fuzzy decision matrix is defuzzied. Then, due to the benefit criteria, the similarity ratio matrix is obtained with equations (
19) and (
20). The similarity ratio matrix is given in Table
A7.
The values in similarity ratio matrix are multiplied by the weights in Table
3 to obtain the weighted similarity ratio matrix. Equation (
22) is used to achieve this objective. The weighted similarity ratio matrix calculated with equation (
22) is expressed in Table
A8.
In
Step 15, geometric means are obtained, and alternatives are ranked. In this scope,
G value is calculated using equation (
23).
G values are demonstrated in Table
5.
Alternatives |
G |
Brazil |
.0973 |
China |
.1031 |
India |
.0990 |
Indonesia |
.0977 |
Mexico |
.0987 |
Russia |
.1033 |
Turkey |
.0977 |
The ranking results of these countries are indicated in Fig.
3.

Fig. 3
Ranking results of the countries.
According to the data presented in Table
5 and Fig.
3, it is evident that Russia occupies the position of the most optimal country. China is the second one in this regard. However, Indonesia and Brazil are the least successful countries with respect to the green communication effectiveness.
4.3 Comparative Analysis with Sine Trigonometric Pythagorean Fuzzy ARAS and Sensitivity Analysis
Step 16 is about comparing the ranking results with two different methods. For this purpose, the results of the ARAS method are obtained using equations (
24)–(
27). For the validity of the results of RATGOS analysis, a second method is used. ARAS method is preferred because it is similar to the optimal value like RATGOS. ARAS method does not include distance metrics like TOPSIS. Since the use of Euclidean distance in ranking alternatives has been criticized in the literature, methods such as MAIRCA, VIKOR, TOPSIS have not been preferred. The decision matrix considered for ARAS is the same as for RATGOS. Here, the weights obtained from DEMATEL are used for the weights of the criteria. Analyses are performed again with the relevant process of the ARAS method. The results of the ARAS method are compared with RATGOS. The comparative results are illustrated in Fig.
4.
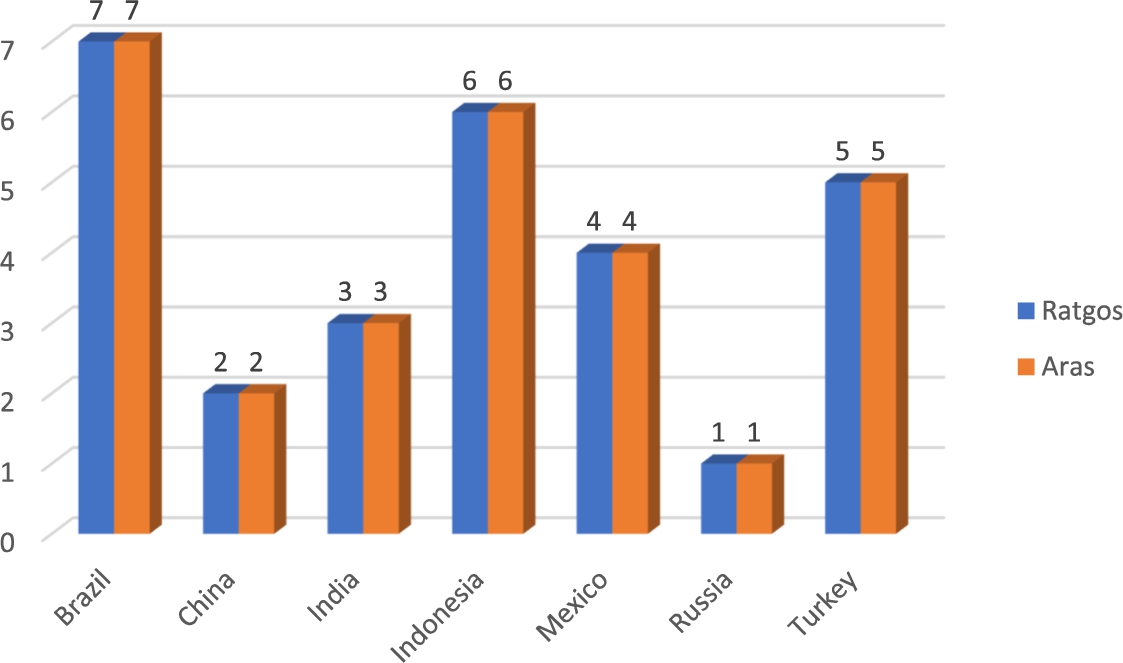
Fig. 4
Comparative analysis results.
Fig.
4 defines that the ranking of the E7 countries remains consistent. In this context, the outcomes of both methods concur, identifying Russia and China as the most favourable countries, with Brazil occupying the lowest position in the rankings. In addition to this situation, a sensitivity analysis is also performed by considering 10 different cases. The details of these results are given in Table
6.
Table 6
Sensitivity analysis results.
|
Case 1 |
Case 2 |
Case 3 |
Case 4 |
Case 5 |
Case 6 |
Case 7 |
Case 8 |
Case 9 |
Case 10 |
Brazil |
7 |
7 |
7 |
7 |
7 |
7 |
7 |
7 |
7 |
7 |
China |
2 |
2 |
2 |
2 |
2 |
2 |
2 |
2 |
2 |
2 |
India |
3 |
3 |
3 |
3 |
3 |
3 |
3 |
3 |
3 |
3 |
Indonesia |
6 |
6 |
6 |
6 |
6 |
6 |
6 |
6 |
6 |
6 |
Mexico |
4 |
4 |
4 |
4 |
4 |
4 |
4 |
4 |
4 |
4 |
Russia |
1 |
1 |
1 |
1 |
1 |
1 |
1 |
1 |
1 |
1 |
Turkey |
5 |
5 |
5 |
5 |
5 |
5 |
5 |
5 |
5 |
5 |
Table
6 indicates that the results of 10 different cases are the same. This situation explains that the proposed model provides coherent and reliable solutions.
5 Discussion
According to the analysis results obtained, the most important issue affecting the success of businesses in the green communication process is informativeness. Green communication refers to the efforts and communication process of businesses regarding environmental sustainability and social responsibility. Informativeness means sharing the elements that constitute the above-mentioned communication in an accurate, transparent, and reliable manner. Customers tend to prefer environmentally friendly businesses. Accordingly, it is important for businesses to provide comprehensive information during the green communication process. In this way, it is possible to increase the brand value of the business as well as ensure customer loyalty. Hsu
et al. (
2022) examined communication approaches for smart cities using renewable energy. In this study, where it is stated that the ideal approach is green communication, the importance of informativeness is mentioned. Vlastelica
et al. (
2023) analysed the factors affecting the environmental and social clothing expenditures of young adults. The study, which used explanatory factor analysis and two-stage structural equation model, was conducted in Serbia with 439 participants. It is underlined that one of the important factors affecting consumer choices is the informativeness of communication. ElHaffar
et al. (
2023) argued that informativeness is a necessary and important condition for communication, especially in cosmetic and personal care product sales.
Production according to the demands and expectations of the target audience is another important factor affecting the green communication process of businesses. The fact that businesses produce in line with their target audience affects the business in many aspects such as profitability and sustainability. This is also very necessary to increase the green communication performance of businesses. Apart from this, customers’ loyalty to the business will increase as the demands and expectations of the target audience are met. Ultimately, it is important to have a strong relationship with the target customer base in order to achieve success in green communication processes. Tewari
et al. (
2022) examined the impact of green communication and openness to change on the purchase intentions of young consumers in India. The importance of the target audience is emphasized in the study, which states that the young population has recently given importance to green approaches. Amoako
et al. (
2022) stated that tourism businesses give more importance to green processes due to customer expectations. It is also emphasized that this creates advantages such as profitability and sustainability. Ellahi
et al. (
2023) underlined that green processes implemented in line with customer demands and needs provide competitive advantage.
As a result of rapid industrialization, developing countries may face some problems such as air pollution. Apart from this, water pollution, intense consumption of natural resources and the size of energy consumption bring along some problems. To reduce the effects of these problems, it is inevitable for E7 countries to give more importance to green processes. Accordingly, in the analysis, the successful countries in green communication among the E7 countries are Russia and China. Ivanchenko
et al. (
2023) stated that the green economy should be developed in Russia to achieve sustainable development goals. In this context, it is stated that Russia attaches importance to green marketing processes. Yuldasheva
et al. (
2023) examined sustainable consumer behaviour models in Russia. It is concluded that Russian consumers have just begun to adopt sustainable consumer behaviour and businesses are making decisions accordingly. Zhao
et al. (
2023) investigated whether there are differences in the effects of green traditional media communication on consumers’ green value creation intentions in post-COVID-19 China. It is identified that China has successfully carried out green processes. Kar and Harichandan (
2022) analysed the studies on sustainable consumption and green marketing in the Scopus and Web of Science databases between 1990 and 2021. Accordingly, China is found as the leader in this regard with 214 total publications.
6 Conclusion
In this study, the determinants of the effective green communication for the companies are evaluated. In this scope, a novel model is proposed that has mainly two different parts. First, indicators are examined with artificial intelligence-based sine trigonometric Pythagorean fuzzy DEMATEL. At the second part, emerging seven countries are ranked regarding the performance of the green communication. For this purpose, artificial intelligence-based sine trigonometric Pythagorean fuzzy RATGOS technique is taken into consideration. On the other side, these countries are also ranked by using ARAS methodology to make a comparative evaluation. It is determined that informativeness is the most significant factor for the improvement of green communication performance of the companies. Moreover, meeting customer expectation is another important situation that should be taken into consideration in this manner. The ranking results of both RATGOS and ARAS are the same. This situation demonstrates that the results of the proposed model are consistent and coherent. It is concluded that China and Russia are the most successful emerging countries with respect to the green communication performance.
The main contribution of this study is the integration of artificial intelligence approach with the fuzzy decision-making methodology. In this context, artificial intelligence methodology is considered to create decision matrix. With the help of this situation, more appropriate calculations can be made. Additionally, proposing RATGOS methodology by the authors is another significant contribution of this proposed model. To overcome criticisms regarding the existing ranking decision-making techniques in the literature, RATGOS model is created by considering geometrical mean in the calculation process. With the help of this condition, it can be possible to reach more effective solutions.
This study does not apply to any sector. This is considered an important limitation of the study. In this context, analysis for a specific sector can be carried out in new studies. For example, green communication is more important for sectors such as agriculture and automotive. In these sectors, it is aimed to attract the attention of customers by introducing environmentally friendly technologies. On the other hand, it is possible to mention some limitations of the proposed model. In the process of integration of artificial intelligence and fuzzy sets, only the working experience of experts is taken into account. Education levels of experts can also be used as a distinguishing factor in future studies. This allows a more comprehensive model to be created. In addition to this issue, DEMATEL methodology is also criticized in the literature. To overcome this problem, a novel decision-making model can be introduced for weighting the criteria.